AI in Cardiology: Where We Are Now and Where to Go Next
From imaging to risk assessment, AI-based tech may free up time for doctoring while improving patient care, but hurdles remain.
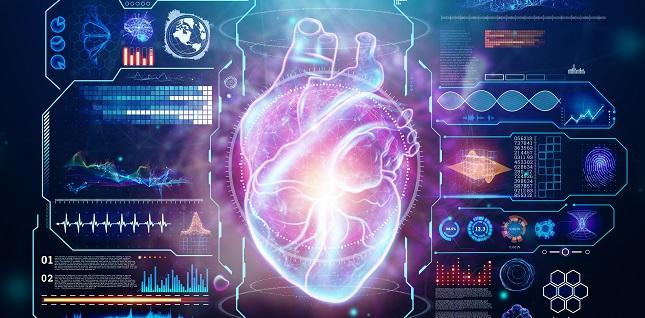
Artificial intelligence (AI) has become a buzzword in every cardiology subspecialty from imaging to electrophysiology, and researchers across the field’s spectrum are increasingly using it to look at big data sets and mine electronic health records (EHRs).
But in concrete terms, what is the potential for AI—as well as its subcategories, like machine learning—to treat heart disease or improve research? And what are its greatest challenges?
“There are so many unknowns that we capture in our existing data sources, so the exciting part of AI is that we have now the tools to leverage our current diagnostic modalities to their extreme to the entirety,” Rohan Khera, MBBS (Yale School of Medicine, New Haven, CT), told TCTMD. “We don't have to gather new data per se, but just the way to incorporate that data into our clinical decision-making process and the research process is exciting.”
“Generally speaking, I'm excited about anything that allows doctors to be more of a doctor and that would make our job easier, so that we can focus our time on the hard part,” Ann Marie Navar, MD, PhD (UT Southwestern Medical Center, Dallas, TX), commented to TCTMD. “If a computer-based algorithm can help me more quickly filter out what therapy somebody should or shouldn't be on and help me estimate the potential benefit of those therapies for an individual patient in a way that helps me explain it to a patient, that's great. That saves me a lot of cognitive load and frankly time that I can then spend in the fun part of being a doctor, which is the patient-physician interface.”
There should not be too much hype, hope, or phobia. Jai Nahar
Although a proponent of AI in medicine, Jai Nahar, MD (George Washington School of Medicine, Washington, DC), who co-chairs the American College of Cardiology’s Advanced Healthcare and Analytics Work Group, said the technology faces hurdles related to clinical validation, implementation, regulation, and reimbursement before it can be readily adopted.
Cardiologists should be balanced in their approach to AI, he stressed to TCTMD. “There should not be too much hype, hope, or phobia. Like any advanced technology, we have to be cognizant about what the technology stands for and how it could be used in a constructive and equitable manner.”
A Foothold in Imaging
Nico Bruining, PhD (Erasmus Medical Center, Rotterdam, the Netherlands), editor-in-chief of the European Heart Journal – Digital Health, told TCTMD there are two main places in hospitals where he sees AI technologies flourishing in the near future: image analysis and real-time monitoring in conjunction with EHRs.
“What we also envision is that some of the measurements we now do in the hospital—like taking the EKG and making a decision about it—can move more to the general practitioner, so closer to the patient at home,” he said. Since it’s already possible to get a hospital-grade measurement onsite, AI will be applied more in primary and secondary prevention, Bruining predicted. “There we see a lot of possibilities, which we see sometimes deferred to a hospital.”
On the imaging front, Manish Motwani, MBChB, PhD (Central Manchester University Hospital NHS Trust, England), who recently co-chaired a Society of Cardiovascular Computed Tomography webinar on the intersection of AI and cardiac imaging, observed to TCTMD that “the field has really exploded” over the past 5 years. “Across all the modalities, people are looking at how they can extract more information from the images we acquire faster with less human input,” he noted.
Already, most cardiac MRI vendors have embedded AI-based technologies to aid in calculating LV mass and ejection fraction by automatically drawing contours on images and allowing for readers to merely make tweaks instead of starting from scratch, Motwani explained. Also, a field called radiomics is digging deeper into images and extracting data that would be impossible for humans to glean.
“When you're looking at a CT in black and white, your human eye can only resolve so much distinction between the pixels,” Motwani said. “But it's actually acquired at a much higher spatial resolution than your monitor can display. This technique, radiomics, actually looks at the heterogeneity of all the pixels and the texture that is coming out with data and metrics that you can't even imagine. And for things like tumors, this can indicate mitoses and replication and how malignant something may be. So we’re really starting to think about AI as finding things out that you didn't even know existed.”
Yet another burgeoning sector of AI use in cardiology is in ECG analysis, where recent studies have shown machine-learning models to be better than humans at identifying conditions such as long QT syndrome and atrial fibrillation, calculating coronary calcium, and estimating physiologic age.
Peter Noseworthy, MD (Mayo Clinic, Rochester, MN), who studies AI-enabled ECG identification of hypertrophic cardiomyopathy, told TCTMD that although “the term ‘low-hanging fruit’ is overused, [this ECG approach] basically readily available data and an opportunity that we wanted to take advantage of.”
Based on his findings and others, his institution now offers a research tool that allows any Mayo clinician to enable the AI-ECG to look for a variety of conditions. Noseworthy said the dashboard has been used thousands of times since it was made available a few months ago. It is not currently available to clinicians at other institutions, but eventually, if it is approved by the US Food and Drug Administration, “then we could probably build a widget in the Epic [EHR] that would allow other people to be able to run these kinds of dashboards.”
“The exciting thing is that the AI-ECG allows us to truly do preventative medicine, because we can now start to anticipate the future development of diseases,” said Michael Ackerman, MD, PhD (Mayo Clinic), a colleague of Noseworthy’s who led the long QT study. “We can start to see diseases that nobody knew were there. . . . AI technology has the ability to sort of shake up all our standard excuses as to why we can't screen people for these conditions and change the whole landscape of the early detection of these very treatable, sudden-death-associated syndromes.”
‘New Era’ of Research
Ackerman believes AI has also opened the door to a “new era” of research. “Every one of our papers combined from 2000 until this year [didn’t include] 1.5 million ECGs,” he said, noting that a single AI study can encompass that level of data. “It's kind of mind-boggling really.”
Bruining said this added capability will allow researchers to “collect the data from not only your own hospital but also over a country or perhaps even over multiple countries. It's a little bit different in Europe than it is in the United States. In the United States you are one big country, although different states, and one language. For us in Europe, we are smaller countries and that makes it a little more difficult to combine all the data from all the different countries. But that is the size you need to develop robust and trustworthy algorithms. Because the higher the number the more details, you will find.”
AI technology has the ability to sort of shake up all our standard excuses as to why we can't screen people for these conditions and change the whole landscape of the early detection of these very treatable, sudden-death-associated syndromes. Michael Ackerman
Wei-Qi Wei, MD, PhD (Vanderbilt University Medical Center, Nashville, TN), who has used AI to mine EHR data, told TCTMD that while “messy,” the information found in EHRs provides a much more comprehensive look at patient cohorts compared to the cross-sectional data typically used by clinical trials. “We have huge data here,” he said. “The important thing for us is to understand the data and to try to use the data to improve the health care quality. That's our goal.”
In order to do that, algorithms must first be able to bridge information from various systems, as otherwise the end result will not be relevant, Wei continued. “A very common phrase in the machine-learning world [is] garbage in, garbage out,” he said.
Siloes and Generalizability
The development of different AI platforms has mostly emerged within institutions, with researchers often validating algorithms on internal data.
That scenario has transpired because to develop these tools, researchers need two things often only found at large academic institutions, said Ackerman. “You need to have the AI scientists—the people who play in the sandbox with all their computational power—and you need a highly integrated, accessible electronic health record that you can then data mine and marry the two.”
Though productive, this system may build in inherent limits to AI algorithms in terms of generalizability.
Motwani pointed to the UK’s National Health System (NHS) to illustrate the point. The NHS, in theory, stores all patient data in a single database, Motwani said. “There's an opportunity for big data mining and you have the database ready to retrospectively test these programs. But on the other hand, in the US you have huge resources but a very fragmented healthcare system and you've got one institution that might find it difficult to get the numbers and therefore need to interact with another institution but there isn't a combined database.”
This has resulted in a situation where much of the data used to develop AI tools are coming only from a handful of institutions and not a broad spectrum, he continued. “That makes it difficult, necessarily, to say, well, all of this applies to a population on the other end of the world.”
Still, “the beauty of AI and machine learning is that it's constantly learning,” Motwani said. “Most vendors, in whatever task they're doing, have a constant feedback loop so their algorithms are always updating in theory. As more sites come onboard, the populations are more diverse that the algorithms are being used on. There's this positive feedback loop for it to constantly become more and more accurate.”
It would be helpful, said Bruining, if there was more open access to algorithms so that they could be more easily reproduced and evolved. “That would help grow interest into these kinds of developments,” he suggested, “because the medical field is still very conservative and not everybody will trust a deep-learning algorithm which teaches itself. So we have to overcome that.”
Navar acknowledged the siloes that many AI researchers are working in, but said she has no doubt that technology will be able to merge and become more generally disseminated with time. “Once an institution learns something and figures something out, [they will be able] to package that knowledge up and spread it in a way that everybody else can share in it,” she predicted, adding that this will manifest in many ways. “There aren't that many different EHR companies in the US market. Although there's competition, there's not an infinite number of technology vendors for things like EKG reading software or echo reading software. So if we're talking about the cardiology space, here this is where I think that there's probably going to be a role for a lot of the companies in the space to adopt and help spread this technology.”
While no large consortium of AI researchers in cardiology yet exists, Bruining said he is petitioning for one within the European Society of Cardiology. For now, initiatives like the National Institutes of Health (NIH)-funded eMERGE network and BigData@Heart in Europe are enabling scientists to collaborate and work toward common goals.
Regulation Speculation
It’s one thing to use technology to help increase efficiency or keep track of patient data, but regulation will be required once physicians start using AI to help in clinical decision-making.
Bruining said many of these AI tools should be validated and regulated in the same way as any other medical device. “If you have software that does make a diagnosis, then you have to go through a whole regulation and you have to inform notified bodies, who will then look. The same thing as with drugs,” he said. “Software is a device.”
Ethically, Khera referred to AI-based predictive models as “a digital assay of sorts” that is no different than any other lab test. “So I think the bar for some of these tools, especially if they alter the way we deliver care, will probably be the same as any other assay,” he said.
Navar agreed. “We're probably going to need to categorize AI-based interventions into those that are direct-to-patient or impacting patient care immediately versus those that are sort of filtered through a human or physician interaction,” she said. “For example, an AI-based prediction model that's used to immediately recommend something to a patient that doesn't go through a doctor, that's like a medical device and needs to be really highly scrutinized because we're depending on the accuracy of that to guide a treatment or a therapy for a patient. If it's something that is augmenting the workflow, maybe suggesting to the physician to consider something, then I think there's a bit more safeguard there because it's being filtered through the physician and so may not be quite as scrutinized.”
Up until now, however, “regulatory approval has not kept pace with these technologies,” Motwani noted, and there lies a challenge for this expanding field. “At the moment, a lot of these technologies are being put through as devices, when actually if you think about it, they're not really.”
Additionally, he continued, many AI-based tools are not prospectively tested but rather developed on historical databases.
“It's very hard to actually prospectively test AI,” Motwani explained. “Are we going to say, ‘Ok, all these X-rays are now going to be read by a computer,’ and then compare that to humans? You'd have to get ethical approval for such a trial, and that's where it gets difficult. The way AI is transitioning at the moment is that it's more of an assistant to human reads. [This] is probably a natural kind of transition that's helpful rather than saying all of a sudden all of this is read by computers, which I think most people would feel uncomfortable with. In some ways, the lack of regulatory clarity is helpful because people are just dipping in and out, they're beginning to get comfortable with it and seeing whether it's useful or not.”
Who Pays?
Who will bear the cost for development and application of these new technologies remains an open question.
Right now, Khera said most tools related to clinical decision support are institutionally supported, although the NIH has funded some research. However, implementation is more often paid for by “health systems in a bid for efficiency in healthcare,” he said. Still other algorithms are covered by the Centers for Medicare & Medicaid Services “like any other assay.”
In the imaging space, Motwani said “most of the companies that are involved are developing fee-to-scan pricing. If you're a large institution and you have a huge faculty, is that really attractive? Probably not. But if you're a smaller institution that maybe doesn't have cardiac MRI readers, for example, it might be cost-effective rather than employing staff to get some of this processing done.”
Yet then questions arise, such as “Are insurers going to pay for this if a human can do it?” and “Is it then that those human staff can then be deployed to other stuff?” Motwani said. “I think the business model will be that companies will make the case that your throughput of cases will be higher using some of these technologies. Read times will be shortened. Second opinions can be reduced. So that's the financial incentive.”
This will vary depending on geography and how a country’s health system is set up, added Motwani. “For example, in the US, the more [scans] that you're doing, the more you're billing, the more money you're making, and therefore it makes sense. Other healthcare systems like the UK where it doesn't work necessarily in that way, . . . there the case would be that you just have to get all the cases done as quickly and effectively as possible, so we'll pay for these tools because the human resources may not be available.”
There are also cost savings to be had, added Bruining. “If you can reduce the workload, that can save costs, and also other costs could be saved if you can use AI more in primary prevention and secondary prevention, because the patients are then coming less to the hospitals.”
Overall, Nahar observed, “once we have robust evidence that this works—it decreases the healthcare spending and increases the quality of care—then I think we'll have more payers buy into this and they'll buy into paying for the cost.”
Fear and Doctoring
The overarching worry about AI replacing human jobs is present in the cardiovascular medicine sector as well, but everyone who spoke with TCTMD said cardiologists should have no anxiety about adopting new technology.
“There's an opportunity to replace an old model,” Wei said. “Yeah, it might replace some human jobs, but from another way, it creates some jobs as well. . . . Machine learning improves the efficiency or efficacy of learning from data, but at the same time more and more jobs will be available for people who understand artificial intelligence.”
Bruining said that technologies come and go, citing the stethoscope as one example of something that has “disappeared more or less” within cardiology. “Physicians and also the nurses and other allied health professionals very much want a reduction in the workload and a reduction in the amount of data they have to type over from one system to another, and that can be handled by AI,” he said.
I think that as humans, we're always going to need the human part of doctoring. Ann Marie Navar
“Is there scope for automation in some of our fields? Definitely so,” said Khera, who recently led a study looking at machine learning and post-MI risk prediction. “But would it supersede our ability to provide care, especially in healthcare? I don't think that will actually happen. . . . I think [AI is] just expanding our horizons rather than replacing our existing framework, because our framework needs a lot of work.”
Navar agreed. “I have yet to see a technology come along that is so transformative that it takes away so much of our work that we have nothing to do and now we're all going to be unemployed,” she said. “I don't worry that computers are going to replace physicians at all. I'm actually excited that a lot of these AI-based tools can help us reduce the time that we spend doing things that we don't need to and spend more time doing the fun or harder part of being a doctor. . . . I think that there's always going to be limitations with any of these technologies and I think that as humans, we're always going to need the human part of doctoring.”
That notion—that AI might allow for deeper connection with patients, rather than taking doctors out of the equation—has been dubbed “deep medicine” after a book of the same name by cardiologist Eric Topol, MD.
COVID-19, Prevention, and Opportunity
In light of the COVID-19 pandemic, Bruining said AI research has been one of the fields to get a bump this past year. “It has torn down a lot of walls, and it also shows how important it is to share your scientific data,” he said.
Motwani agreed. “On a quick Google search, you'll find hundreds and hundreds of AI-based algorithms for COVID,” he said. “I think the people who had the infrastructure and databases and algorithms rapidly pivoted toward the COVID. Now whether they've made a difference in it prospectively is probably uncertain, but the tool set is there. It shows how they can actually use that data for a new disease and potentially if there were something to happen again.”
Looking forward, Nahar sees the largest opportunity for AI in prediction of disease and precision medicine. “Just before the disease or the adverse events manifest, we have a big window for opportunity, and that's where we could use digital biomarkers, computational biomarkers for prediction of which patients are at risk, and target early intervention based on the risk intervention to the patient so they would not manifest their disease or the disease prevention would be halted,” he said. “They would not go to the ER as frequently, they would not get hospitalized, and the disease would not advance.”
Navar said for now cardiologists should think of AI more as “augmented” intelligence rather than artificial, given that humans are still an integral part of its application. In the future, she predicted, this technology will be viewed through a different lens. “We don't talk about statistics as a field. We don't say, ‘What are the challenges of statistics?’ because there's like millions of applications of statistics and it depends on both where you're using it, which statistical technique you're using, and how good you are at that particular technique,” she said. “We're going to look back on how we're talking about AI now and laugh a little bit at trying to capture basically an entirely huge set of different technologies with a single paintbrush, which is pretty impossible.”
Yael L. Maxwell is Senior Medical Journalist for TCTMD and Section Editor of TCTMD's Fellows Forum. She served as the inaugural…
Read Full BioDisclosures
- Wei, Bruining, Khera, Noseworthy, Motwani, and Nahar report no relevant conflicts of interest.
- Ackerman and Mayo Clinic have an equity/royalty relationship with AliveCor Inc but without remuneration thus far.
- Navar reports receiving personal fees from Cerner Corporation and JAMA Cardiology.
Comments