AI Shows Potential for Predicting New-Onset AF from ECGs
The next step is to test artificial intelligence to identify patients at risk for AF as a means of preventing related stroke, authors say.
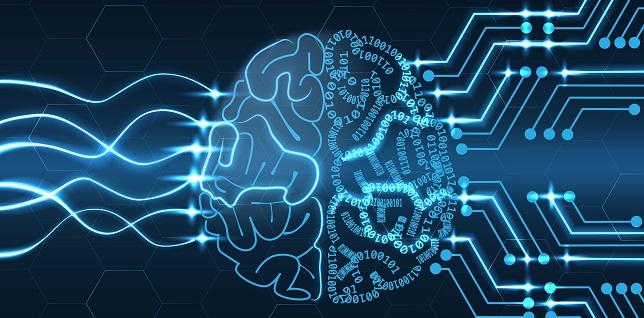
A novel artificial intelligence (AI) model can predict new-onset atrial fibrillation (AF) among patients with no history of the condition, potentially improving the possibility of identifying those at risk for AF-related stroke down the road, according to a new study.
“The risk of stroke with atrial fibrillation doesn't always require previous symptoms—it can be a presenting symptom in a significant number of cases. So, the ability to screen atrial fibrillation early is a definitive benefit,” senior author Christopher M. Haggerty, PhD (Geisinger Medical Center, Danville, PA), told TCTMD.
With the recent onslaught of studies showing the potential for machine learning in the field of cardiology—from identifying long QT syndrome to predicting coronary calcium—this technology is poised to change practice.
“We've thought about the possibility for this type of model to be a resource for a health system,” Haggerty said. “A cardiology practice within a health system such as Geisinger could be systematically running ECGs that are collected through this type of model to identify within an entire population who are the patients who are at risk and then respond accordingly to try to help maximize the utility and the health of a population as a whole.”
For the study, published online Tuesday ahead of print in Circulation with lead author Sushravya Raghunath, PhD (Geisinger Medical Center, Danville, PA), the investigators used 12-lead ECG data from 430,909 patients with no history of AF collected between 1984 and 2019 to train (80% of ECGs) and validate (20%) a deep neural network. The ECGs used for validation were obtained between 2010 and 2014 and linked to a stroke registry.
The model was able to predict new-onset AF within 1 year with an area under the receiver operator curve of 0.85 (95% CI 0.84-0.85) in the training set and 0.84 set (95% CI 0.83-0.85) in the validation set. This metric increased slightly when including only AF presenting between 1 and 31 days from the baseline ECG (0.87; 95% CI 0.86-0.88).
The risk of actually developing AF over 30 years was higher for those in whom the model predicted a high versus low risk of new-onset AF within 1 year (HR 7.2; 95% CI 6.9-7.6).
Additionally, the model predicted new-onset AF at 1 year with a sensitivity of 69% and specificity of 81%, with a number needed to screen to find a single new case of AF of 9. Lastly, of the 375 patients who reported an AF-related stroke within 3 years of their index ECG, the model estimated a high risk for new-onset AF in 62%.
Next Steps
Haggerty said he was pleased with how the model performed in the training cohort, but the true impact will come from their real-world deployment scenario looking at the patients who went on to have AF-related strokes. “Extrapolating from that, here's a use case where you can have ECGs from patients that can be processed through this model and, if predicted to be high-risk, the patient might be subjected to some more-intensive follow-up screening to identify if and when A-fib in fact develops. And that identification may help them to get on a lifesaving medication to prevent the development of stroke,” he said.
It remains to be seen then how that type of enhanced screening for atrial fibrillation can actually impact stroke outcomes. Christopher Haggerty
Other work done by researchers using AI to mine ECG data has been encouraging and has reinforced “that we're onto something here,” Haggerty said. As a next step, they plan to conduct a prospective ambulatory monitoring study to further validate their model. “Of course, it remains to be seen then how that type of enhanced screening for atrial fibrillation can actually impact stroke outcomes. [It’s] a broader question that I know many in the field are trying to answer.”
Lastly, while the focus of this study was to prevent AF-related stroke by early identification of new-onset AF, the researchers write that a model of this kind could be useful also for predicting things like arrhythmia-induced cardiomyopathy. “In addition to allowing early treatment for new-onset AF, a clinical risk prediction tool such as this could be used for the prevention of AF,” they say. “A high-risk prediction of future AF could bring increased attention to modifiable risk factors such as obesity and obstructive sleep apnea, with the goal of avoiding AF altogether.”
Yael L. Maxwell is Senior Medical Journalist for TCTMD and Section Editor of TCTMD's Fellows Forum. She served as the inaugural…
Read Full BioSources
Raghunath S, Pfeifer JM, Ulloa-Cerna AE, et al. Deep neural networks can predict new-onset atrial fibrillation from the 12-lead electrocardiogram and help identify those at risk of AF-related stroke. Circulation. 2021;Epub ahead of print.
Disclosures
- The study was supported in part by funding from the Geisinger Clinic and Tempus Labs. Geisinger receives funding from Tempus for ongoing development of predictive modeling technology and commercialization. Tempus and Geisinger have jointly applied for a patent related to the work.
Comments