AI Shows Promise in Detecting Type 1 MI and Need for Revascularization
AI outperformed clinicians and almost matched hs-TnT testing, but one expert questioned if this is where it’s needed most.
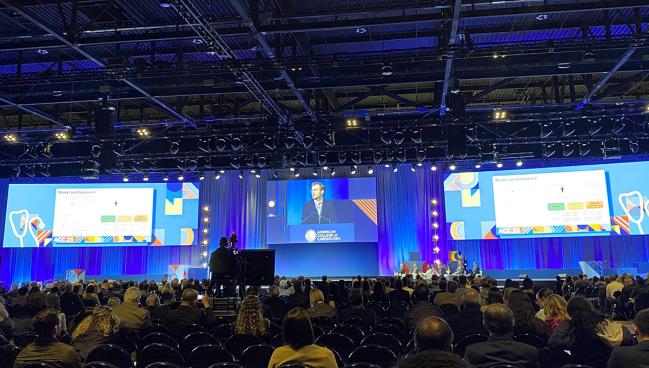
CHICAGO, IL—A novel artificial intelligence (AI)-powered tool can detect type 1 MI using ECG data better than expert clinicians and with about the same level of accuracy as a high-sensitivity troponin T (hs-TnT) assay, according to a new analysis.
The deep-learning model was trained on almost 200,000 ECGs from two international cohorts of patients presenting to the emergency department (ED) with NSTE ACS, and the results suggest clinicians may one day get AI help when moving appropriate patients from the emergency department to the cath lab, say investigators.
In patients without ST-segment elevation, ECG patterns can be “heterogeneous and nonspecific,” leading to variability in clinician interpretation, said lead investigator Antonius Büscher, MD (University Hospital Münster, Germany), adding that high-sensitivity troponin testing helps but can take time and result in false positives.
“All these factors significantly complicate the diagnostic process and increase uncertainty in the emergency setting,” said Büscher last week during a late-breaking clinical trials session at the American College of Cardiology 2025 Scientific Session. “Our aim was to leverage technology to reduce uncertainty by automatically recognizing ECG patterns that predict patients’ need for revascularization.”
In the study, published simultaneously online in the European Heart Journal, data from 180,686 patients (mean age 60 years; 53% female) treated at Beth Israel Deaconess Medical Center in Boston, MA, between 2008 and 2022 were used to train (80%) and test (20%) the model, and data from 18,673 patients (mean age 55 years; 49% female) from University Hospital Münster were used to externally validate it. Coronary revascularization rates were 0.6% in the US population and 1.5% in the German cohort.
When it came to predicting which patients required revascularization in the test population, the model outperformed clinicians’ interpretation of the ECG and conventional hs-TnT testing (AUROC 0.91, 0.65, and 0.71, respectively). Results were similar in the external validation cohort, with the ECG-AI model outperforming clinicians for interpretation, although it was inferior to the predictive performance of hs-TnT (AUROC 0.81, 0.70, and 0.85, respectively).
For the diagnosis of spontaneous MI (type 1), the AI model was better than clinicians’ interpretation of the ECG, but not as good as when hs-TnT was used (AUROC 0.85, 0.74, 0.87, respectively).
Büscher expected a drop in the model’s performance in the external validation cohort as this is “normal” when AI algorithms are applied to a new population using ECG data from different machines as well as variations in clinical practice. But it’s ability to outperform clinicians and almost match hs-TnT was “encouraging because it suggested that the model had genuinely learned to recognize features that are associated with myocardial ischemia,” he added.
“In fact, when we looked closer into how the model made its decisions, we confirmed that it relied on well-known ischemic changes like ST-segment and T-wave alterations. So essentially, the model has rediscovered features that we as clinicians already recognize as ischemic, but it was able to identify these features more precisely and correlate them more effectively with clinical outcomes,” Büscher said.
Understanding the ‘Black Box’
Discussing the study during the session, Thomas Maddox, MD (WashU Medicine, St. Louis, MO), said the results reinforce that “artificial intelligence in clinical cardiology is here.” The next step will be to better define standards for achieving consistent quality and safety with these innovations going forward, he said.
Maddox called AI’s ability to see patterns within an ECG that a clinician cannot a bit of a “black box,” adding that “we need to somehow have faith that what the machine is telling us represents the biological reality of what we’re trying to uncover.” The study findings are reassuring in this regard, he argued, given how the researchers explained the model’s ability to connect ECG variations with clinical outcomes.
With up to 900,000 cases of ACS treated annually in the US, Wayne Batchelor, MD (Inova Heart and Vascular Institute, Fairfax, VA), said it is imperative to find ways to better treat this “huge public health issue.”
Addressing the media during a press briefing, he said an AI model “conceptually” makes sense to aid in this regard but might not be the most practical given that standard care involves hs-TnT testing for patients within 1-3 hours of arrival to the ED with chest pain.
“In my mind, I’m still left trying to understand what’s the incremental value in the model over top of what we really do in the contemporary care, which is EKG, one troponin, another troponin, and decision to go the cath lab,” he said. “These patients probably, at least as what we know so far, do not need to go extremely urgently. . . . The questions are going to be: is it worth having this extra deep-learning model look at EKGs and how much incremental value is there over the normal treatment of the patient?”
Yael L. Maxwell is Senior Medical Journalist for TCTMD and Section Editor of TCTMD's Fellows Forum. She served as the inaugural…
Read Full BioSources
Büscher A, Plagwitz L, Yildirim K et al. Deep learning electrocardiogram model for risk stratification of coronary revascularization need in the emergency department. Eur Heart J. 2025;Epub ahead of print.
Disclosures
- Büscher and Maddox report no relevant conflicts of interest.
Comments