AI Tool Fails to Boost Outcomes in ED Triage of Suspected CV Conditions
The RAPIDx AI trial did show the clinical decision support tool could improve some key metrics of management.
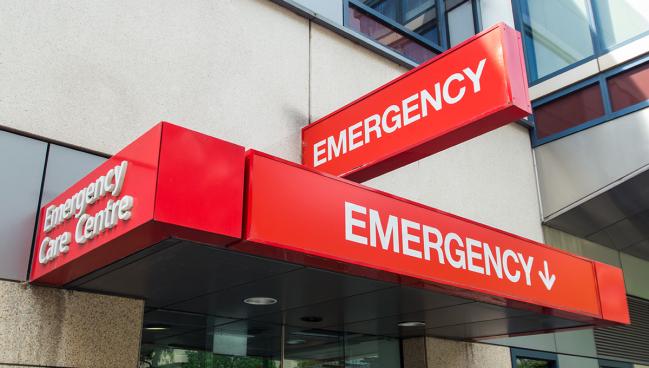
Hospitals whose emergency department (ED) triage of patients with suspected cardiac conditions was informed by an artificial intelligence (AI)-based clinical support tool saw no improvement in clinical outcomes, according to the RAPIDx AI trial.
That’s not to say there were no benefits, however. The AI tool, by identifying or ruling out type 1 MI, appeared to help clinicians tailor the use of medical therapy and angiography.
Derek Chew, MBBS, PhD (Victorian Heart Hospital, Clayton, Australia), presenting the results recently at the European Society of Cardiology Congress 2024 in London, England, pointed to the central roles of diagnosis and risk stratification in providing evidence-based ACS care.
While the Fourth Universal Definition of MI (UDMI) can tease out whether patients have myocardial infarction or myocardial injury of nonischemic origin, “delivering this at scale in emergency departments everywhere—where there may not be experts or 24/7 across an entire health service—is really the challenge we need to meet these days given our workforce changes,” he said in a Hot Line session on the conference’s final day.
“Meeting this promise, artificial intelligence potentially gives us the opportunity to deliver this therapy, this intervention, across an entire health service, and therefore is potentially the most disruptive technology of our era,” added Chew.
The RAPIDx AI investigators set out to design an AI tool aligned with the Fourth UDMI to see whether having that available at a hospital level would impact outcomes.
As it turns out, the effect was modest, Chew told TCTMD via email. “This may be due to us targeting decision-making at the ED, rather than at the cardiologists.”
Building and Testing the AI Interface
To start, the RAPIDx AI researchers developed their AI tool by using machine learning (XGBoost/Deep Learning) to analyze regional registry data contained in electronic health records. This process was informed by clinical characteristics at the time of index ED presentation as well as subsequent outcomes. They tested two algorithms that differentiated type 1 MI from other forms of myocardial injury. One was related to the choice to admit or safely discharge patients, while the other pinpointed various phenotypes (type 1 MI, type 2 MI/acute injury, or chronic injury).
Then, for the cluster-randomized trial, Chew and colleagues analyzed data on 14,131 adults who presented to the EDs of six metropolitan and six rural hospitals in South Australia over an 8-month period in 2023. The approach to triage was randomized at a hospital level: six centers in the control arm with unchanged standard of practice and six in the intervention arm with access to AI-based clinical decision support via an interface (Siemens) that offered recommendations on medical therapy, further testing, and invasive management.
Ultimately, 3,029 patients (mean age 74.5 years; 58% female) were found to have myocardial injury based elevated high-sensitivity cardiac troponin with suspected cardiac cause: 48% had presented to control hospitals and 52% to AI-informed hospitals.
In this intention-to-treat population, the primary endpoint—a composite of CV death, new/recurrent MI, and CV readmission within 6 months—was nearly identical between the control and intervention groups, at 26.4% and 26.0%. In the overall cohort, the rates were 10.4% and 9.4%, respectively. The safety endpoint of all-cause death or MI within 30 days among those directly discharged from the ED was noninferior for patients who presented to AI-equipped hospitals compared with those who presented to control hospitals (0.86% vs 1.1%; P for noninferiority < 0.001).
For patients classified as not having type 1 MI (n = 2,441), invasive coronary angiography was less likely with AI-informed care than with usual care (5.2% vs 9.5%), as were revascularization and beta-blocker use. Patients who were classified as having type 1 MI (n = 578), on the other hand, had higher rates of being prescribed statin therapy (82% vs 68%), P2Y12 inhibitor therapy (56% vs 44%), and a mineralocorticoid receptor antagonist (26% vs 18%) in the AI arm compared with the control arm, but there were no differences in invasive management or revascularization.
An exploratory analysis that excluded STEMI patients, for whom management would be informed by ECG and likely not involve the input of AI, found a significant difference in the rate of CV death/MI favoring the intervention hospitals over controls (HR 0.81; 95% CI 0.66-0.99).
Reassuringly, the AI-identified phenotypes also tracked well with patients’ clinical outcomes.
“Machine-learning models are able to differentiate those patients with myocardial injury who have a higher risk of recurrent MI, and therefore appear to benefit from the early invasive strategy, [meaning that] they can help clinicians differentiate patients who should and should not be referred for coronary angiography,” Chew explained to TCTMD.
While making these insights available to clinicians didn’t change much about management in RAPIDx AI, this doesn’t bring an end to their work, said Chew. “We will continue to slowly educate clinicians on the improved clinical discriminatory capacity of machine learning in clinical care and consider reengineering workflows to improve translation of the diagnostic insights.”
But What About the Doctor?
On the heels of Chew’s ESC presentation, the assigned discussant was circumspect. “I was impressed by your study and by this artificial intelligence database,” Barbra Backus, MD, PhD (Franciscus Gasthuis Hospital, Rotterdam, the Netherlands), commented. “However, I'm also a bit wondering about how that good old doctor [fits in].”
She questioned why such an intricate approach was necessary, given that so many risk calculators—such as TIMI, EDACS, and HEART—already exist. “I'm actually pretty confident that a combination of a doctor and a risk stratification tool is very good in our risk prediction,” said Backus, providing data to demonstrate her point. “I really think that we should not forget how well we are trained and how well our clinical gestalt can [inform] our decision-making.”
Session chairperson Nicholas Mills, MBChB, PhD (University of Edinburgh, Scotland), noted how hard it must have been to perform RAPIDx AI. “Step-wedge trials are difficult. Deploying AI tools into clinical practice is a challenge, and to do that in multiple emergency departments across countries is a huge endeavor,” said Mills, adding, “I'm sure it's going to be the first of many trials evaluating these sorts of tools that we'll see over the next decade. So really important [that] we learn from what went well and what didn't go so well from trials like this.”
The way the trial was designed from a hospital perspective, though, makes it hard to know how the AI support was used at a clinician level,” he pointed out. “So how did you track whether the clinician was engaging with the tool in order to ensure that the guideline recommendations that you made were adhered to?”
“That's the challenge,” Chew agreed.
“Many of us think this might be a trial of an algorithm, but it's not. . . . We’ve struggled with this issue of evidence translation in ACS care and in many other parts of medicine for an eternity,” he observed. “This is really about how, where the rubber hits the road, whether or not clinicians engage with the insights that are presented to them to be able to deliver expert-level care.”
For RAPIDx AI, the solution to this conundrum existed in the platform itself. “The clinician, to get the insight, needed to interact with the tool . . . that Siemens helped us build,” said Chew. The trial has collected details on when and exactly how clinicians received information, as well as on the care they provided. These analyses are forthcoming, he said.
In his email to TCTMD, Chew stressed that “machine learning and AI are here to stay” and merit ongoing, careful study. It won’t be easy, he acknowledged.
“Translating [the technologies] in practice requires sophisticated data architecture, and investing in this at scale is hard to do.”
Caitlin E. Cox is News Editor of TCTMD and Associate Director, Editorial Content at the Cardiovascular Research Foundation. She produces the…
Read Full BioSources
Chew D. RAPIDx AI: re-engineering the clinical approach to suspected cardiac chest pain assessment in the emergency department by expediting evidence to practice using artificial intelligence. Presented at: ESC 2024. September 2, 2024. London, England.
Disclosures
- Chew reports research contracts with NHMRC Australia, SA Health, and Siemens Healthineers.
Comments