Machine Learning Helps Predict In-Hospital Mortality Post-TAVR, but Skepticism Abounds
This type of artificial intelligence produced an accurate model. Is it clinically useful?
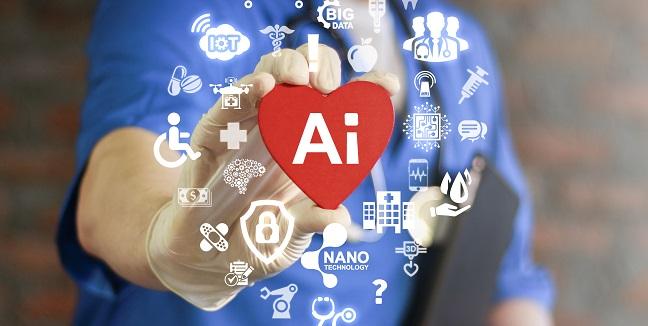
(UPDATED) Machine learning, a subset of artificial intelligence (AI), may help stratify risk of in-hospital mortality following TAVR, a new analysis shows. However, critics are questioning whether the approach studied has any useful clinical applications.
Four different models developed through machine learning were deemed to have good performance for predicting risk of in-hospital mortality in a cohort of patients undergoing TAVR in the United States, with the best one derived using logistic regression, Dagmar Hernandez-Suarez, MD (University of Puerto Rico School of Medicine, San Juan), and colleagues report.
“The good discrimination of this model reveals the potential of AI in the patient risk stratification process, not just for TAVR but for any novel structural intervention,” they write in their paper published online ahead of the July 22, 2019, issue of JACC: Cardiovascular Interventions. “Further validation and application of machine learning into the day-to-day clinical practice is still warranted to better understand its true value in patients with severe aortic stenosis.”
Because the models were built using both preprocedural and postoperative factors, Hernandez-Suarez told TCTMD “the clinical utility of the developed prediction algorithm is mainly directed to prognosis.” That could be useful for letting patients and their families know what the likelihood of surviving to discharge is in the event of a complication, he said, pointing out that the usefulness of this tool for patient selection is limited.
Commenting for TCTMD, David J. Cohen, MD, MSc (University of Missouri-Kansas City), was critical of the study, pointing in particular to one serious limitation involving how the models were developed. Because they include postoperative complications occurring during the hospitalization up until the time of discharge, the models can be run only after patient discharge, at which point it’s already clear whether the patient died, he said.
Where we’ve seen machine learning start to make an impact in medicine is in things like image processing, where there’s just so much information that the human mind cannot handle the complexity. David J. Cohen
“The model can’t be run properly until you know about both the presence and the absence of those complications, but you don’t know about the absence of a complication until the patient has left the hospital,” Cohen said. “The purpose the authors are describing is potentially valid, but the model they have developed cannot actually address that issue.”
Cohen also questioned the value of a tool like this one to assess whether a patient will survive to discharge. “Most models developed to support shared decision-making in interventional cardiology are designed to be implemented prior to the procedure,” he said.” This model was designed to predict in-hospital prognosis after the procedure and any associated complications have occurred. At that point, the only shared decision-making to be done is to decide whether to withdraw care from a patient, which seems to be of limited utility.”
What Is Machine Learning?
Though various tools have been developed in recent years to help assess risk of in-hospital mortality in patients being considered for TAVR, “these models have only relied on conventional statistical methods, which carry inherent limitations that might affect its application and performance in large data sets with multiple variables and samples,” Hernandez-Suarez et al say.
Machine learning represents a different approach. Hernandez-Suarez described it as “a discipline of computer science or artificial intelligence that basically focuses on predicting outcomes of complex data sets using algorithms that iteratively learn from data. So far, machine learning has been demonstrated to be very useful in the generation of robust prediction models.”
He and his colleagues applied machine learning to information taken from the National Inpatient Sample database on 10,883 patients (mean age 81; 48% women) who underwent TAVR between January 2012 and September 2015. Overall, 3.6% of patients died prior to discharge.
The four different models were developed using logistic regression, artificial neural network, naive Bayes, and random forest algorithms. The researchers report that all had “good” performance as measured by the area under the receiver-operating curve (AUC). The model based on logistic regression came out on top (AUC 0.92), followed by the naive Bayes (AUC 0.90), random forest (AUC 0.90), and artificial neural network (AUC 0.85) models.
Performance for all increased as up to 10 variables were introduced, after which there was little improvement in the logistic regression and naive Bayes models and some decline in performance in the other two.
The most heavily weighted factors across models, in order of importance, were acute kidney injury, cardiogenic shock, fluid and electrolyte disorders, cardiac arrest, sepsis, hyperlipidemia, hypertension, coagulopathy, current smoking, and vascular complications.
Hernandez-Suarez acknowledged that there is some additional work that needs to be done before the models developed in this study will reach clinical utility. He pointed to the need for external validation in other cohorts of TAVR patients and further evaluation using more recent data that would reflect the shift toward greater use of TAVR in lower-risk patients.
But for now, Hernandez-Suarez said, “I think this study actually has set the benchmark for the use of machine learning in structural cardiology.”
Cutting Through the Hype
In an accompanying editorial entitled, “Machine Learning Is Not Magic: A Plea for Critical Appraisal During Periods of Hype,” Thomas Modine, PhD (Hôpital Cardiologique, CHRU de Lille, France), and Pavel Overtchouk, MD (University Hospital of Bern, Switzerland), take a cautious approach in discussing the potential applications of machine learning, both in this study and in a broader sense.
Interpretability, say Modine and Overtchouk, remains a major limitation of predictive models based on deep learning, “a branch of machine learning that uses deep neural networks,” and their implementation in practice.
“As of now, the numerous intertwined relationships captured by the layers of a deep neural network are only partially understood, leading to their frequent labeling as ‘black boxes’ and the observed trade-off between the accuracy and interpretability of machine learning models,” they explain. “Given the importance of a doctor’s ability to explain the exact reasons that led to a medical decision, understanding the functioning of deep neural networks is important to correct their malfunctions, including their bias and susceptibility to slight modifications of analyzed data.”
It’s really critical that we incorporate our medical bioinformatics specialists, our statisticians, the AI experts if we want to integrate artificial intelligence to make our lives easier and to make the way in which we manage and adjudicate patients’ risk more holistic and accurate. Rishi Puri
As research into machine learning and deep learning continues, the editorialists say, “more effort should be made by researchers and authors to explain their models, describing their processes, behavior, and estimations, with the help of graphical representations, and providing code when appropriate.” And, they add, medical journals should bolster their expertise to handle what is likely to be a growing number of papers like this.
Cohen agreed, saying that the fact that this study was published in a high-quality journal after peer review highlights an important issue. “In order to truly provide high-quality peer review for a study like this one, we need both methodologists who are experienced in the analytic techniques (ie, machine learning), but we also need content experts who understand what they’re trying to answer and the limitations of the data that they’re using, to put the whole story together.”
Rishi Puri, MD, PhD (Cleveland Clinic, OH), made a similar point. “It’s really critical that we incorporate our medical bioinformatics specialists, our statisticians, the AI experts if we want to integrate artificial intelligence to make our lives easier and to make the way in which we manage and adjudicate patients’ risk more holistic and accurate,” he commented to TCTMD.
He concurred with Cohen that the approach studied by Hernandez-Suarez and colleagues has limited clinical utility. But, he added, “having said that, it is to our knowledge the first risk score that is using an artificial intelligence or machine learning type of methodology. And perhaps that is why the manuscript was well received by the journal. It’s not necessarily because we need to agree with their risk score and throw the STS score under the bus. Not at all. Sometimes manuscripts are accepted and published to create awareness.”
AI is here to stay and will be increasingly integrated into daily practice, Puri said. “I think moving forward the sky is the limit in terms of how AI can help us . . . so I think the purpose of this manuscript really is to raise awareness to get people talking.”
Taking a broader view, Cohen said machine learning has potential for clinical use, but the greatest opportunity may be in other settings. “Where we’ve seen machine learning start to make an impact in medicine is in things like image processing, where there’s just so much information that the human mind cannot handle the complexity. That’s where machine learning really shines,” Cohen commented.
In their editorial, Modine and Overtchouk end with a note of caution. “[R]eaders should remember that critical appreciation of research papers is vital, especially for topics subject to hype. Statistical significance does not guarantee clinical pertinence. Excellent results reported in ‘innovative’ studies must be verified in independent studies with different cohorts and be subjected to the test of time. Given the performance of machine learning and deep learning approaches, their foreseen implementation in health care is likely. And as the hype passes, real applications should start to appear.”
Todd Neale is the Associate News Editor for TCTMD and a Senior Medical Journalist. He got his start in journalism at …
Read Full BioSources
Hernandez-Suarez DF, Kim Y, Villablanca P, et al. Machine learning prediction models for in-hospital mortality after transcatheter aortic valve replacement. J Am Coll Cardiol Intv. 2019;12:1328-1338.
Modine T, Overtchouk P. Machine learning is no magic: a plea for critical appraisal during periods of hype. J Am Coll Cardiol Intv. 2019;12:1339-1341.
Modine reports being a consultant for Boston Scientific, Medtronic, Edwards Lifesciences, Cephea, Microport, GE, and Abbott; and having received a research support grant from Edwards Lifesciences.
Disclosures
- The study was funded by the US National Institutes of Health.
- Hernandez-Suarez and Overtchouk report no relevant conflicts of interest.
Comments