Medicaid Coverage Improves CV Risk in Some, Post Hoc Analysis Shows
Machine learning-based techniques identified a subset who had a meaningful reduction in BP when they received coverage.
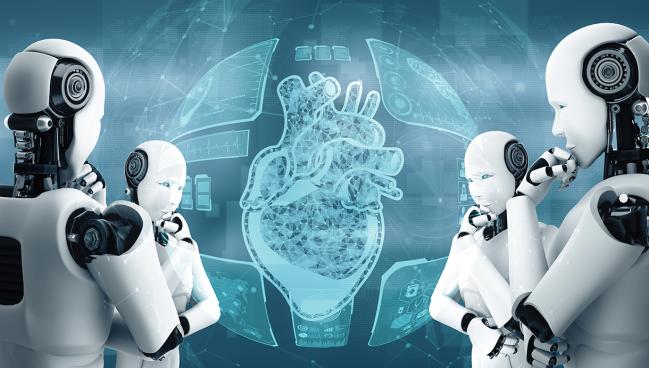
Providing Medicaid coverage is likely to lead to meaningful health benefits for at least a subset of previously uninsured, low-income Americans, according to a post-hoc analysis of the randomized Oregon health insurance experiment.
Obtaining Medicaid through a lottery system led to a significant reduction in systolic blood pressure among those identified through machine learning-based techniques as having a high likelihood of benefit, contrary to the lack of change observed in the main results of the study, which was launched back in 2008, lead author Kosuke Inoue, MD, PhD (Kyoto University, Japan), and colleagues report in a paper published recently in the BMJ.
“This study used a newer machine learning approach to find out who gets a larger benefit from Medicaid coverage than others, and we did find some heterogeneity in terms of how much health benefit each individual in the data experienced by having Medicaid coverage,” senior author Yusuke Tsugawa, MD, PhD (David Geffen School of Medicine at UCLA, Los Angeles, CA), told TCTMD.
He added, “The average null effect of the Medicaid coverage in the original study was probably because the true benefits incurred by subgroups were masked by other people who did not experience improvement in physical health by having Medicaid coverage.”
Insights From Machine Learning
There are few randomized trials that have evaluated the impact of insurance coverage on health outcomes, Tsugawa said. One of them, the Oregon health insurance experiment, involved a statewide lottery that would determine who could sign up for Medicaid coverage because there wasn’t enough funding for everyone. Some measures of health were improved, with increases in the diagnosis and treatment of diabetes and use of some preventive services, a decrease in the probability of screening positive for depression, and a marked reduction in catastrophic out-of-pocket medical expenses. But there was no significant effect on CV risk factors like systolic BP and glycated hemoglobin (HbA1c).
That was puzzling to policymakers and health policy researchers, Tsugawa said, because insurance coverage was expected to improve health.
In the current analysis, the investigators applied machine learning to identify subsets of patients with either a high or low likelihood of benefit from Medicaid coverage to explore heterogeneity in the effects. “These novel techniques can identify complex heterogeneous effects across many potentially intertwined variables that are not shown by the conventional stratified analysis,” they explain, adding that the study aims “to provide a more nuanced understanding of how Medicaid coverage might influence cardiovascular risk factors.”
The analysis included 12,134 adults (mean age 41 years; 57% women) who lived in Oregon, had an income below the federal poverty line, and were previously uninsured. At baseline, mean systolic BP was 119 mm Hg and mean HbA1c was 5.3%.
Overall, neither metric was influenced by Medicaid coverage, but by applying a machine learning causal forest algorithm with instrumental variables, the investigators identified individuals with a high likelihood of benefit. In this subset (about three-quarters of the overall trial population for BP), getting coverage with Medicaid significantly lowered systolic BP (-4.96 mm Hg vs -0.62 mm Hg in the overall trial cohort) and diastolic BP (-3.91 mm Hg vs -1.00 mm Hg; P < 0.001 for both).
Though HbA1c also was significantly reduced in the high-benefit group (about 59% of the study cohort for this measure), the difference compared with the overall study population was not clinically meaningful (-0.12% vs 0; P = 0.009), the researchers report.
The participants who had a high expected benefit from gaining Medicaid coverage tended to be those with little or no prior spending on healthcare while those who had a low expected benefit had higher prior spending, possibly through safety-net hospitals or federally qualified health centers, despite being uninsured, Tsugawa pointed out.
“They were getting treatment and diagnosis even before getting insurance, so that was not surprising to us,” he said. But, he added, this wasn’t “one of the things that the investigators looked into in the original health insurance experiment. Using this novel machine learning approach gave us a richer understanding of what was happening at the time when this RCT was conducted.”
Methodological Implications
Commenting for TCTMD, Jason Wasfy, MD (Massachusetts General Hospital, Boston), who has studied the impact of Medicaid expansion on cardiac care, said this analysis is interesting from a methodological perspective.
“These types of applications of machine learning algorithms with causal interpretations can help us figure out if there are specific populations that benefit more from treatments than other populations,” he said.
That may be less important in the realm of insurance coverage than in other areas of clinical medicine, Wasfy indicated. “As a practical matter, there's broad consensus in healthcare about the idea that all patients should have access to care, which requires all patients to have insurance.”
These novel methods, however, could be applied in secondary analyses of other types of trials to help tailor care, he suggested. “It's very interesting to think about the application of machine learning to trial results that are either showing a small effect or a negative effect and to use those methods to tease out subpopulations that may benefit.”
Tsugawa noted that most trials include analyses of only a limited number of subgroups. “The machine learning approach can use a large number of covariates to investigate who benefits more from the intervention, and this is also what we think of when we talk about precision medicine.”
For instance, these techniques could be used to predict the benefits and harms of a particular treatment before administering it, he suggested. “And I think a wider use of this kind of approach can advance the field and advance our clinical understanding of who should get a treatment versus not, and why.”
In an accompanying editorial, Chengliang Yang, MD, and Scott Tebbutt, PhD (both from St. Paul’s Hospital, University of British Columbia, Vancouver, Canada), touch on the broader implications of these results, saying, “The application of machine learning techniques in health policy research enables the identification of varying treatment effects across different groups, showing insights that traditional methods might miss. While artificial intelligence encompasses machine learning, machine learning is specifically useful for such targeted analysis.”
Todd Neale is the Associate News Editor for TCTMD and a Senior Medical Journalist. He got his start in journalism at …
Read Full BioSources
Inoue K, Athey S, Baicker K, Tsugawa Y. Heterogeneous effects of Medicaid coverage on cardiovascular risk factors: secondary analysis of randomized controlled trial. BMJ. 2024;386:e079377.
Yang C, Tebbutt SJ. Effects of Medicaid coverage on cardiovascular health outcomes. BMJ. 2024;386:q1807.
Disclosures
- The study was supported by the Japan Society for the Promotion of Science, the Japan Science and Technology Agency, the National Institutes of Health (NIH), and Gregory Annenberg Weingarten, GRoW @ Annenberg.
- Inoue reports funding from the Japan Agency for Medical Research and Development and the Program for the Development of Next generation Leading Scientists with Global Insight (L-INSIGHT) sponsored by the Ministry of Education, Culture, Sports, Science and Technology (MEXT), Japan, for other work not related to this study.
- Tsugawa reports receiving funding from the NIH/National Institute on Aging and the NIH/National Institute on Minority Health and Health Disparities for other work not related to this study and serving on the board of directors of M3, Inc.
- Yang and Tebbutt report a research grant from the Canadian Institutes of Health Research.
Comments