New Machine Learning-Based Tool Predicts Individual Post-PCI Outcomes
The researchers polled patients to see what they would find most meaningful in a predictive risk calculator and implemented it.
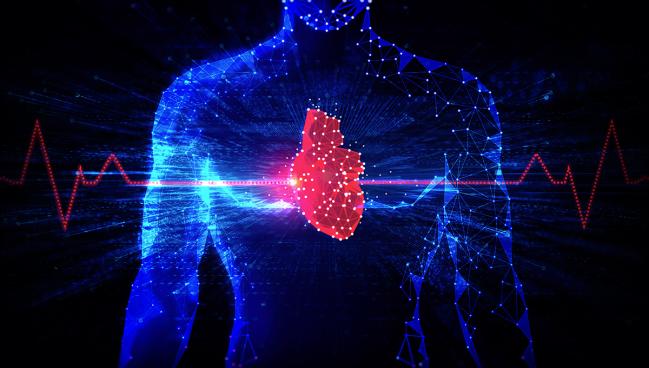
A novel machine learning-based algorithm can predict individualized risk of death and complications after PCI, as demonstrated in a new analysis.
The Blue Cross Blue Shield of Michigan Cardiovascular Consortium (BMC2) learning models showed robust accuracy for predicting the risk of not only in-hospital mortality but also acute kidney injury (AKI), new-onset dialysis, stroke, major bleeding, and transfusion.
“Not only is this something that is good for administrative functions and identifying patients' risk in general, but also [it adds a] personal touch and something that can really impact conversations with patients,” lead author David E. Hamilton, MD (University of Michigan, Ann Arbor), told TCTMD.
The individual outcomes included in the algorithm were selected after surveying 66 patients about their preferences for what the model would display.
“We had assumed—and I think the assumptions are kind of wrong—that patients would want to hear about the most serious complications, but the patients pushed back and said the risk importance varies on personal perspective,” senior author Hitinder S. Gurm, MBBS (University of Michigan), told TCTMD. “They felt that it was important that we provide all the risk numbers and the patient can decide what is the important number for them, not try to classify them as major and minor as is normally done in many papers, clinical trials, and other areas.”
In an accompanying editorial, Patrick W. Serruys, MD, PhD (University of Galway, Ireland), and colleagues laud the researchers for creating a “patient-centered tool” to further the field of personalized medicine. “Another noteworthy aspect of this study is its focus on individual outcome prediction, a departure from creating composite outcomes. This strategic choice enhances the interpretability and actionable nature of the outcome risk predictions,” they write.
High Discrimination Value
For the study, published online last week in the European Heart Journal, Hamilton, Gurm, and colleagues first used data from 107,793 adults in the BMC2 registry (mean 67 years old; 68.1% female) who underwent PCI at one of 48 hospitals in Michigan between April 2018 and December 2021 to train and validate their algorithm using machine learning-based eXtream Gradient Boosting (XGBoost) models.
In this population, the overall incidence of in-hospital mortality was 1.85%, AKI was 2.51%, new-onset dialysis was 0.44%, stroke was 0.41%, major bleeding was 0.89%, and need for transfusion was 2.41%.
The XGBoost models performed with excellent discrimination for mortality with an area under the curve (AUC) of 0.930 as well as AKI (AUC 0.893), dialysis (AUC 0.951), transfusion (AUC 0.917), and major bleeding (AUC 0.887). Stroke, which was the least frequent outcome for this cohort, demonstrated modest discrimination with an AUC of 0.751.
The researchers then validated their tool using the population of 56,583 adults from the Cardiac Care Outcomes Assessment Program (COAP) database who underwent PCI at one of 33 hospitals in Washington during the same period. Risk prediction from the XGBoost models on this data set was consistent with the excellent discrimination seen in the BMC2 cohort, including moderate risk discrimination for stroke.
To further personalize their tool, the researchers polled an initial patient advisory panel of five who had previously undergone PCI to offer “qualitative assessments of their personal shared decision-making process and quantitative rank order importance assessment of post-PCI adverse events.” The resulting survey was taken by 66 patients, of whom 67% were women, 85% were white, and 85% had a bachelor’s degree. Most respondents (64%) requested the tool to display all postprocedural risks individually as opposed to grouped as composite endpoints.
Improving Upon What’s Available
Gurm explained that the goal with this tool was to merge multiple outcomes into one prediction tool, unlike other similar projects have been able to do thus far. Additionally, he said, the BMC2 models are enhanced as not only are they validated by multiple populations, but also they factor in the personalized data that patients really want to see.
“The performance of this model is just exceptional,” he said. “And I don't use that term lightly, but it was great in our population and [the Washington] population. That, to me, gives me a lot of confidence that our results are really robust.”
Acknowledging that some outcomes are easier to predict than others, Gurm said that he wasn’t surprised to see the tool wasn’t as robust at predicting stroke because it occurs less frequently and it is “also dependent on patient anatomy factors that are not captured in these databases. . . . The model didn't do that well simply because we don't have the data elements that might help.” The next iterations of these models will likely include data from patient images and potentially improve prediction that way, he added.
“The systematic screening approach employing machine learning has the potential to unravel novel and nontraditional critical prognostic factors in the context of randomized trials or registries,” Serruys and colleagues write. “Utilization of ‘big data’ for individual decision-making undoubtedly offers substantial benefits to patients. However, it is important to acknowledge that the machine learning process can be challenging to visualize the decision process and weight of each factor, and, while it consistently demonstrates high performance, it requires ongoing scrutiny and validation by clinicians to ensure its accuracy.”
Next, Hamilton said he’d like to see the models continue to be tested, perhaps in an international cohort. Also, he’d like to see the model eventually include longer-term outcomes “because PCI and coronary disease is certainly not something that just ends when you leave the hospital,” he said.
Gurm highlighted that they will make their model “freely available” to clinicians and researchers to use and further validate and are open to feedback about how to improve it.
Yael L. Maxwell is Senior Medical Journalist for TCTMD and Section Editor of TCTMD's Fellows Forum. She served as the inaugural…
Read Full BioSources
Hamilton DE, Albright J, Seth M, et al. Merging machine learning and patient preference: a novel tool for risk prediction of percutaneous coronary interventions. Eur Heart J. 2024;Epub ahead of print.
Serruys PW, Kageyama S, Onuma Y. Cardiology’s new crystal ball: machine learning for outcome prediction. Eur Heart J. 2024;Epub ahead of print.
Disclosures
- Hamilton reports no relevant conflicts of interest.
- Gurm reports receiving research support from Blue Cross and Blue Shield of Michigan paid to his institution. He is the co-founder of, owns equity in, and is a consultant to Amplitude Vascular Systems. He also owns equity in Jiaxing Bossh Medical Technology Partnership and is a consultant for Osprey Medical. He is the chair of the Clinical Events Committee for the PERFORMANCE trial sponsored by Contego Medical.
- Serruys reports receiving consulting fees from SMT, Novartis, Merillife, Philips, and Xeltis.
Comments