AHA Sums Up AI’s Potential in Cardiology, but Also the Hurdles Ahead
Building trust in these tools through prospective data as well as proper labeling might enable them to make a clinical difference.
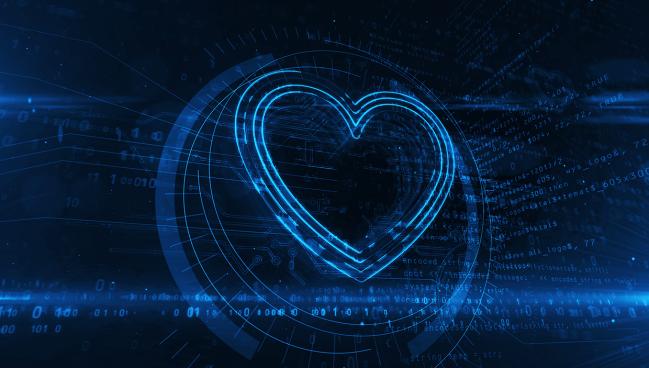
Questions about the impact of artificial intelligence (AI) on cardiovascular medicine should be qualified with a “when” rather than “if,” according to a new American Heart Association (AHA) scientific statement. Much work remains, though, before these tools can be widely trusted to improve patient care, the authors stress.
Outlining the ways AI, machine learning, and deep learning have already been engrained in medical practice as well as the tools in development, the authors express optimism about their potential to improve diagnosis and treatment as well as prevention, but had some words of caution. “Despite enormous academic interest and industry financing, AI-based tools, algorithms, and systems of care have yet to improve patient outcomes at scale,” the authors state.
“As with any technology, we get excited about its impact, but I believe this is a technology where the impact is unknown,” writing committee chair Antonis Armoundas, PhD (Massachusetts General Hospital, Boston, MA), told TCTMD.
Armoundas stressed the commitment of physicians to “do no harm” and pointed out that improper use of AI-based tools has the potential to adversely affect patients.
“It feels like an Oppenheimer moment as we are trying to seek out how to improve outcomes for our patients, whether these are healthcare outcomes or quality of life,” he explained. “The speed at which this technology evolves makes us humble in being able to ground ourselves and think of the implications of what we are trying to accomplish, how we are going to achieve these goals, and being mindful of the potential negative effects that it could have.”
The statement, published online last week in Circulation, is the AHA’s second addressing AI this year, with the first directed specifically at its role in cardiac imaging.
What we should be seeking in the future is to build trust for these technologies, as with every other use of technology in medicine. Antonis Armoundas
With a wide variety of AI-based algorithms now available, including for reducing cath lab activation time in STEMI, detecting cardiomyopathy in pregnancy, and identifying heart failure or hypertrophic cardiomyopathy, the impact of these tools is already being felt by cardiologists. In compiling a statement of best practices and associated challenges, Armoundas said the AHA statement aimed to focus both on what’s worked as well as identifying gaps and challenges, providing a framework for future efforts.
From clinicians to researchers, IT executives, and government entities, he said all invested stakeholders can take something away from the statement. “This manuscript aims to provide a motive: a reason to go deeper and to look for more issues of interest,” Armoundas said.
Best Practices and Associated Challenges
The authors identify six main uses and clinical applications of AI within the field of cardiology: cardiac imaging, electrocardiology, continuous bedside monitoring, mobile and wearable technologies, genetics, and electronic health records (EHR). Along with best practices for each of these categories, they list specific gaps and challenges as well. The biggest ones surround patient safety and data protection, bias and fairness, accountability and reliability, regulations and liability, cybersecurity and system upgrades, and clinical decision-making.
With in-hospital monitoring, for example, remote sensors may help improve the accuracy of alarms as well as reduce alarm fatigue. However, the authors point out that while this might sound appealing, limited data exist for these tools and the research that has been done shows that their effect can be altered by patient behavior.
Additionally, they cite the potential for AI to mine EHR data to make diagnoses and predict outcomes like in-hospital mortality. Again, though, challenges around EHR data curation and consistency have been shown to directly affect the potential for AI-based tools in this space, and the authors advise waiting until those issues are corrected before putting any algorithm into routine practice.
As exciting as many of these algorithms sound, Armoundas cautioned that there is a broad shortage of prospective data at this time, and among the studies that do have prospective designs, many are limited by narrow demographics. Increasing the generalizability of these algorithms will give these tools the chance to have a greater impact, he said. “What we should be seeking in the future is to build trust for these technologies, as with every other use of technology in medicine.”
This can only be done gradually, Armoundas continued, through prospective clinical trials. But the US Food and Drug Administration will also play a role in the way it labels these tools for use. “If an algorithm is used ‘as labeled’ by the FDA, perhaps that would provide the level of security and the level of trust when it is used by clinicians and when it has to be adopted by patients,” he said, adding that this will be especially important as these tools start to be used in broader populations of patients than those in the initial studies.
Another issue, he explained, is how physicians can best incorporate their own opinions with the algorithm output when making clinical decisions. “We argue that algorithms at this point are more likely to be used in conjunction with expert clinician opinion, albeit we do have evidence today, especially in imaging studies, that an algorithm can perform better than an expert clinician,” Armoundas said. “Going back to the point of using an algorithm on an as-labeled basis, that provides not only guidance to clinicians, but provides also a level of comfort in terms of liability.”
Assigning a level of probability to these algorithms will also be imperative for incorporating them into clinical care so that clinicians can make informed judgements on how to act on the data provided, he added.
Keep an Eye on AI
In a commentary published on the AHA’s Professional Heart Daily website, Caroline Marra, PhD, Joseph B. Franklin, JD, PhD, and Amy P. Abernethy, MD, PhD (all from Verily Life Sciences; South San Francisco, CA), write that “though there is growing consensus on the need for adequate monitoring of AI tools, agreement on the right level of monitoring is lacking and figuring out how to accomplish monitoring across so many domains is a daunting challenge.”
They argue for the creation of infrastructure to be able to simultaneously analyze multiple data sources but also acknowledge that thus far efforts to do this have “generated more questions than answers.”
Marra et al conclude that “AI tools provide an incredible opportunity to enable continuous improvement, innovation, and equity in our healthcare systems” and hold the potential to optimize health for all, with the caveat that this will only be possible and responsibly done if the performance of AI tools can be tracked as they’re deployed in practice.
Yael L. Maxwell is Senior Medical Journalist for TCTMD and Section Editor of TCTMD's Fellows Forum. She served as the inaugural…
Read Full BioSources
Armoundas AA, Narayan SM, Arnett DK, et al. Use of artificial intelligence in improving outcomes in heart disease: a scientific statement from the American Heart Association. Circulation. 2024;Epub ahead of print.
Marra C, Franklin JB, Abernethy AP. How should we think about monitoring the effect of AI-based tools on clinical outcomes? Published on: February 28, 2024. Accessed on: March 7, 2024.
Disclosures
- Armoundas reports no relevant conflicts of interest.
- The commentary authors report employment and equity ownership in Verily Life Sciences.
- Abernathy reports stock ownership in EQRx, Georgiamune, One Health, and Iterative Health as well as consulting fees from Sixth Street and ClavystBio.
Comments