AI-ECG Allows for Early Diagnosis of Low EF
Randomized data show potential for a clinically integrated algorithm: the trick will be actually putting information to use.
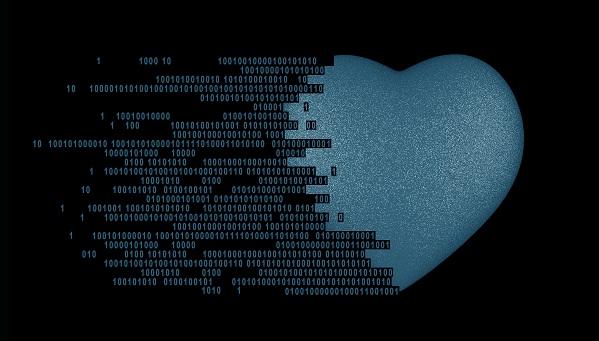
An artificial intelligence (AI)-enabled ECG algorithm integrated into routine care can increase the diagnosis of low ejection fraction (EF), according to new randomized trial data.
“Because ECG is a low-cost test frequently performed for a variety of purposes, the algorithm could potentially improve early diagnosis and treatment in broad populations,” write Xiaoxi Yao, PhD (Mayo Clinic, Rochester, MN), and colleagues in their study published online last week in Nature Medicine. The algorithm uses neural networks to predict a high likelihood of low EF, an often-missed predictor of adverse events, based on standard 12-lead electrocardiogram data.
But the success of the technology—developed by the same team who recently published results on identifying long QT syndrome in a similar fashion—is dependent on it actually being used by clinicians, Yao told TCTMD. Since a myriad of AI-based algorithms are created daily, and money and IT resources to be engaged, “we cannot afford to implement all the AI algorithms into the EHR [electronic health record],” she said, noting the importance of randomized trials in this space.
EAGLE Findings
For the EAGLE study, Yao and colleagues cluster randomized 120 primary care teams in Minnesota and Wisconsin to treat patients either with access to their AI-ECG, which gives an indication of potential for low EF (n = 181 clinicians), or with usual care (n = 177 clinicians).
Overall, 11,573 patients without prior heart failure were assessed via the intervention pathway and 11,068 served as controls between August 2019 and March 2020. Six percent of patients in each group had positive AI-ECG results, indicating a high likelihood of low EF.
More echocardiograms were obtained for patients with positive AI-ECG results in the intervention arm compared with patients whose clinicians did not have access to the technology (49.6% vs 38.1%; P < 0.001). However, echocardiogram use was similar for the cohorts in the overall population (19.2% vs 18.2%; P = 0.17).
Additionally, clinician access to AI-ECG data increased the diagnosis of low EF compared with usual care, both overall (2.1% vs 1.6%; OR 1.32; 95% CI 1.01-1.67) and especially among patients with positive results (19.5% vs 14.5%; OR 1.43; 95% CI 1.08-1.91).
The effect of the intervention was consistent across a spectrum of subgroups, with the exception that it increased the diagnosis of low EF to a greater degree among patients who received their ECGs in outpatient clinics.
“The AI-enabled EKG facilitated the diagnosis of patients with low ejection fraction in a real-world setting by identifying people who previously would have slipped through the cracks,” senior author Peter Noseworthy, MD (Mayo Clinic), said in a press release.
Yao said the most surprising study finding to her was that even with a positive AI-ECG result, “about half of the time clinicians didn't order an echo.” She said they have since surveyed clinicians to dig deeper into their rationale—data that will be published at a later date—but speculated several reasons why this might have been the case. “Some patients might not be able to afford the cost, some patients might have other competing priorities, or clinicians just don't think it's valuable in this context,” she said, adding that some clinicians simply don’t believe they need AI to tell them what to do.
Broader Benefit
In addition to giving insight into the specific use and effect of this AI-algorithm, the authors say the study offers broader benefit.
“The trial was conducted across diverse practice settings, including primary care clinics at a tertiary medical center as well as community and rural clinics,” they write. “The clinicians involved in the study were not AI experts or specialists but were busy clinicians engaged in the care of patients presenting with a wide range of conditions. Therefore, this trial might form a template for how new technologies can be studied in ways that closely mirror their potential deployment.”
Also, this type of study can save costs and time, the authors say. “This pragmatic trial was embedded in everyday practice to efficiently generate relevant and valid evidence. The study design, embedding of the technology in the EHR, and enrollment and follow-up was completed in less than 2 years at a much lower cost than a traditional RCT. Given the increasing number of AI tools being developed, this framework might facilitate rigorous and timely evaluation and implementation.”
“The AI itself is the intervention to be evaluated, but the AI can also help the clinical trials to be done fairly quickly,” Yao added. “During the trial, we also evaluated implementation strategies and identified the problems so we can fix them in the subsequent implementation. So, once the trial is done, it can be quickly turned to a translation or used for implementation.”
Yael L. Maxwell is Senior Medical Journalist for TCTMD and Section Editor of TCTMD's Fellows Forum. She served as the inaugural…
Read Full BioSources
Yao X, Rushlow DR, Inselman JW, et al. Artificial intelligence-enhanced ECG identifycation of low ejection fraction: a pragmatic, cluster-randomized clinical trial. Nature Med. 2021;Epub ahead of print.
Disclosures
- The study was funded by Robert D. and Patricia E. Kern Center for the Science of Health Care Delivery.
- Mayo Clinic has licensed the AI-ECG algorithm to EKO, a maker of digital stethoscopes with embedded ECG electrodes. At no point will Mayo Clinic benefit financially from the use of the AI-ECG for the care of patients at Mayo Clinic.
- Yao reports no relevant conflicts of interest.
Comments