AI Poised to Change the Landscape of Echocardiography
Two studies presented at AHA show the capacity of AI to improve sonographer accuracy, consistency, and workflow.
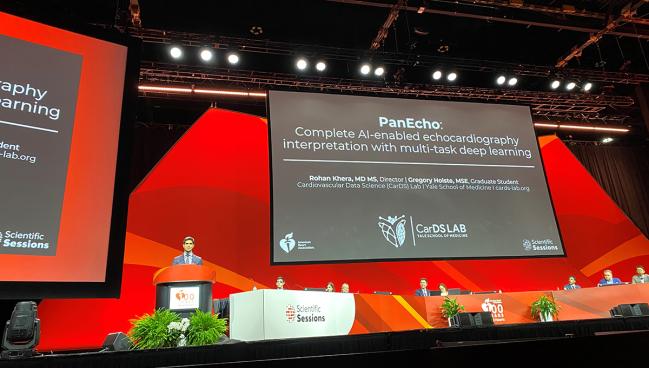
CHICAGO, IL—Artificial intelligence (AI) has already changed the way echocardiography is practiced, but two new studies presented here at the American Heart Association 2024 Scientific Sessions show its potential to transform the landscape even further.
“Echocardiography is the ideal place to use AI,” David Ouyang, MD (Cedars-Sinai Medical Center, Los Angeles, CA), said during a press conference. “It’s the most common form of cardiac imaging. It’s hundreds to thousands of times more common than CT and MRI. It covers the full spectrum of disease, meaning that we use it in both very sick patients as well as very healthy patients for screening because it’s cheap, there’s no radiation, and it’s portable.”
Intraobserver variability as well as the potential for lower-quality images in certain patient groups are the “Achilles’ heel” of this imaging modality, however, he said.
Randomized studies of AI in the field of echocardiography have been few and far between, with PROTEUS and EchoNet standing out. Observational studies have shown machine learning’s potential to improve efficiency in generating echo reports and to improve the accuracy with which novice sonographers image patients with heart failure.
Of the studies presented here, the first was a randomized trial showing that an AI-based workflow could improve sonographer efficiency and output while reducing mental fatigue compared with conventional scanning. The second was a proof-of-concept study of a new AI tool that can comprehensively perform whole heart echocardiography with a high degree of accuracy.
Commenting on the new research for TCTMD, Akhil Narang, MD (Northwestern University Feinberg School of Medicine, Chicago), said two of the major challenges the field of echocardiography is facing include the increased complexity of the images without the proper expertise needed to read them as well as a “major shortage” of sonographers.
AI is poised to help with both issues. “Echo really becomes ripe for the picking when it comes to artificial intelligence,” he said. “I think these two studies potentially help in some of those regards.”
AI-Echo Benefits Sonographers
Nobuyuki Kagiyama, MD, PhD (Juntendo University, Tokyo, Japan), presented data from the AI-ECHO randomized controlled trial. The researchers randomized four Japanese sonographers to perform scans on a daily basis either with their usual technology—performing traditional manual measurements—or AI assistance—using the Us2.ai system (Singapore) to automatically calculate almost 70 parameters, verifying the measurements as needed. Echo physicians checked and approved all reports.
Over a study period of 38 days, the sonographers read a total of 268 and 317 scans on non-AI and AI days, respectively. Cases were well balanced between the groups with regard to patient sex (54% and 60% female), mean age (64 and 65 years), and body mass index (22.9 and 23.2 kg/m2), and most echocardiographic parameters were within normal ranges with minimal cardiovascular disease.
Compared with the standard process, the AI workflow reduced the examination time (14.3 vs 13.0 minutes; P < 0.001) and increased the number of daily exams (14.1 vs 16.7; P = 0.003). AI assistance also increased the number of echocardiographic parameters analyzed (25 vs 85; P < 0.001). The investigators found “very high concordance” of the AI’s initial values and those in the final report, Kagiyama said.
Sonographers, when surveyed about their use of the system, said that AI assistance significantly reduced mental fatigue; investigators also saw a trend toward reduced physical fatigue despite the increased case load. “This workflow enhancement may enable sonographers to spend more time on human-centered and clinically enriching tasks, potentially boosting job satisfaction,” Kagiyama suggested.
Lastly, blinded reviewers determined that the rate of excellent image quality was higher on AI days compared with non-AI days. “This may be because sonographers did not have to measure parameters and could focus more on image acquisition,” according to Kagiyama. “Another possibility is that, as the AI’s accuracy depends on image quality, sonographers aimed to take better images to ensure the AI provided accurate measurements.”
While it’s notable that the AI system decreased scan times, Ouyang pointed out that this Japanese group had “very fast sonographers.” US sonographers typically take 45 to 60 minutes to scan a single patient, he said.
Narang agreed that there’s a big difference between current Japanese and US echo practice. “We usually earmark about an hour per scan per patient, and so sonographers are usually scanning about seven to eight patients a day depending on how long their shifts are,” he said. “I think already, the Japan groups probably do limited echos, and it certainly shows that they can do more echos with this with shorter time to acquire those images.”
It’s all a balance, however, Narang said, as it not only takes time to image patients and conduct the measurements, but there’s also mental and physical effort involved in prepping patients and getting them settled on the table, answering questions along the way.
“I think that it remains to be seen where [AI-echo] is worth it” and where it has clinical applicability, he said, adding that he’d like to see future replication studies.
PanEcho Assessment
The second analysis, co-presented by Rohan Khera, MD, MS, and PhD candidate Gregory Holste (both Yale School of Medicine, New Haven, CT), tested the ability of a novel AI tool called PanEcho, a system designed to fully automate echo analysis and interpretation.
“In many settings right now, the main bottleneck to cardiovascular healthcare is actually limited access to these highly skilled personnel,” Holste said during a media briefing. “This is where there’s a real opportunity for AI to automate echo analysis. However, so far, these efforts have only automated one task at a time, often using images from one view at a time.”
PanEcho was trained on 1.2 million echocardiography videos, then subsequently evaluated for accuracy in reading 39 parameters.
Across 18 diagnostic tasks, PanEcho achieved a median area under the receiver operating characteristic curve (AUROC) of 0.91, particularly excelling at ventricular assessment. The researchers also found the system could accurately estimate continuous echocardiographic parameters with a median normalized mean absolute error of 0.13, predicting LVEF within 4% and estimating LV posterior wall thickness within 1.2 mm on average.
“Critically, PanEcho’s performance on each of these tasks is comparable with existing state-of-the-art AI models that are specialized for each task, whereas PanEcho performs all of these simultaneously,” Holste said.
An additional analysis found the AI tool maintained accuracy across all 18 tasks when it was limited to five key views (median AUROC 0.85). “This signals a potential use case for PanEcho-assisted screening in point-of-care settings where complete echocardiograms cannot be feasible due to limited time or expertise,” he noted.
Lastly, the researchers externally validated PanEcho using two populations from California where it showed “strong performance across geography,” according to Holste.
“This can change our echocardiographic workflows, both for the standard echo lab to provide a pre-read, and also at the core lab to provide a new layer of standardization for echocardiography in the patient,” Khera added.
The researchers have made the full model including code architecture available online for anyone interested in testing PanEcho in their institution.
“This is really what happens when you introduce computer scientists [to] cardiologists,” Ouyang commented. “It allows for really exciting new technologies to be developed.”
He further applauded Khera and Holste for releasing their code, observing that this will give others the chance to build upon what they’ve already accomplished with PanEcho. “There’s a lot of infrastructure required to build this model, and a lot of this is not fully spoken of in such a presentation because it’s a lot of background work,” Ouyang added.
Narang said that while the PanEcho technology is “quite good,” it “would not substitute for echocardiographers, but maybe provide an extra layer of pre-reading, which I think is welcome.” AI could also help ensure the quality and reliability of the echo report, he added. “It’s kind of this idea that the human plus the AI together will be better than the human alone.”
Future Potential
Though many tout the potential of AI to improve echocardiography in resource-limited hospitals, Ouyang told TCTMD that for now, it’s likely going to be embedded more into echo labs at academic centers before becoming more widely available.
“Unfortunately, it’s still a place of ‘the rich get richer,’” he said. “The easiest place to implement will be in the echo lab because it actually has a common workflow, it’s relatively standardized, and that’s where the images they got to train the model came from. In the point-of-care setting, many physicians don’t save their images, they might not have access to the internet that actually allows running these models, and fundamentally, the images are of lower image quality. So it’s harder for the AI to assess.”
Low-resource settings would undoubtedly benefit from AI systems like the ones studied here, but their ability to do so will depend on their capacity to acquire the images in the first place, Narang said. “But the bigger use case I think can be in large-scale centers that have a lot of patients and a lot of volume,” he continued, noting that implementation of this technology into the existing infrastructure will be the trickiest part.
Narang said his lab has already been testing the Us2.ai system to see if it will be a good fit and would be interested in testing out PanEcho. This process “requires all stakeholders to come to the table—the echocardiographers, the sonographers, and administrators,” he said. “The other thing to pay attention to is cost. Is there a return on investment for the cost of this technology?”
While all of these new tools are exciting and progress will continue, Narang urged clinicians to be ready to embrace AI, but also conduct due diligence. “There are a lot of vendors out there, and only a few will emerge as the ones that are the viable ones for long-term integration and financial sustainability.”
Yael L. Maxwell is Senior Medical Journalist for TCTMD and Section Editor of TCTMD's Fellows Forum. She served as the inaugural…
Read Full BioSources
Kagiyama N. Artificial intelligence-based automated echocardiographic measurements and the workflow of sonographers: randomized crossover trial (AI-Echo RCT). Presented at: AHA 2024. November 16, 2024. Chicago, IL.
Khera R, Holste G. PanEcho: Complete AI-enabled echocardiography interpretation with multi-task deep learning. Presented at: AHA 2024. November 16, 2024. Chicago, IL.
Disclosures
- Kagiyama reports serving as a speaker for Novartis Japan, Nippon Boehringer Ingelheim, Otsuka Pharmaceutical Company, and Eli Lilly Japan and receiving research funding from Bristol Myers Squibb and AstraZeneca.
- Khera reports receiving research funding from Bristol Myers Squibb, BridgeBio, and Novo Nordisk and having an ownership interest in Ensight-AI and Evidence2Health.
- Holste reports no relevant conflicts of interest.
- Ouyang reports serving as a consultant to Invision and receiving research funding from AstraZeneca and Alexion.
Comments