AI Tool Can Quantify CAC—and Associated CV Risk—From PET
Once in clinical use, the model can save patients from additional radiation exposure and reduce costs, Piotr Slomka says.
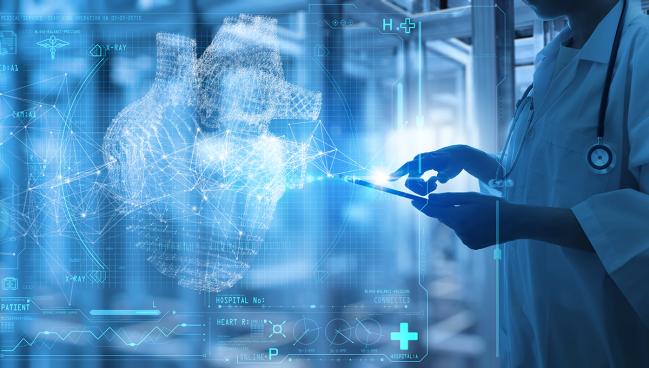
A novel deep learning-based model can, by using low-quality positron emission tomographic (PET)/computed tomography (CT) scans, automatically quantify coronary artery calcium (CAC) and its potential for causing future adverse cardiac events, according to new data.
Patients undergoing PET/CT imaging are typically being evaluated for myocardial perfusion or other noncardiac purposes, but the authors say these scans can serve a dual purpose.
“Every single one of these perfusion scans, which comes with an attenuation map, actually can have a calcium score which prognostically seems to be equivalent with these scores, which are obtained from separate additional scans,” senior author Piotr J. Slomka, PhD (Cedars-Sinai Medical Center, Los Angeles, CA), told TCTMD. “This can reduce the cost and streamline utilization of a calcium score.”
Equivalent to Standard Estimation
For the study, published online today ahead of print in JACC: Cardiovascular Imaging, Slomka, lead author Konrad Pieszko, MD, PhD (Cedars-Sinai Medical Center), and colleagues created and trained a deep-learning model to recognize and quantify coronary calcium from CT attenuation correction (CTAC) scans that always accompany PET imaging. They trained the model on 9,543 expert-annotated CT scans (mean age 65 years; 53% male) and tested in in 4,331 patients (mean age 71 years; 58% male) from an external cohort undergoing PET/CT imaging with 4.3 years of MACE data, including same-day paired ECG gated CAC scans for 2,737 patients.
The model their tool is based on—called convolutional long-short term memory—was originally developed for nonclinical video applications like facial recognition.
In fewer than 6 seconds per scan, the deep-learning model was able to stratify patients by MACE risk across four CAC categories— 0, 1-100, 101-400, and > 400—with a stepwise increase identified (HR up to 3.2; P < 0.001).
There was no significant reclassification improvement found in CAC when the standard scores were individually evaluated compared with when the model automatically estimated them (-0.02; 95% CI -0.11 to 0.07). Also, for patients with zero CAC, the negative predictive values for MACE were similar for standard evaluation and the deep-learning model (85% vs 83%; P = 0.19).
‘A Fuller Picture’
Slomka said that, going in, he expected the tool to work, as similar technology has already shown success in measuring plaque volume and stenosis severity from coronary CT angiography (CCTA).
“However, what's especially novel in this study here and what surprised me a little bit is that the prognostic information we found by this fully automatic method on those very-low-quality scans is essentially the same as prognostic information obtained by expert clinicians on those high-end, gated standard calcium scans,” he said. “Despite this fully automated approach, we're able to basically show the same risk stratification.”
Because the model was trained and tested with several different cohorts from different centers, Slomka said it is “really well validated.” His team is taking steps to apply the model to every patient who undergoes PET/CT imaging at their institution.
Currently, the data from CTAC scans are “thrown out basically . . . or only visually estimated by the physicians,” Slomka observed. “I think every [one] of these PET/CT scans could be quantified with such methods and that additional information—that deep-learning calcium score obtained from these low-quality attenuation maps—could be given to the physician to consider.”
From there, he clarified that they are not recommending a change to what might happen if a standard CAC scan identified prognostically relevant calcium.
“We're just saying that maybe you don't have to do this extra additional scan; save the radiation, save the cost,” Slomka said. “Because the physician right now, they would maybe order that calcium scan separately to be sure, but then it's not really reimbursable right now. Of course, there is a debate about this, but if that CT scan is reimbursable and already that information is there, perhaps that facilitates for the patient to have it available without this extra cost and radiation.”
It’s possible that other helpful clinical information is lurking in CTAC scans, and Slomka said this should be the focus of future research.
“By having automatic AI software running in the background and quantifying these features—of course, physicians will review it, they can see that on the image exactly where the computer found these lesions, they can correct it, they can ignore it, they can delete it—but by [having a] computer finding this extra additional information, I think it can provide a fuller picture of the patient's health,” he said.
Yael L. Maxwell is Senior Medical Journalist for TCTMD and Section Editor of TCTMD's Fellows Forum. She served as the inaugural…
Read Full BioSources
Pieszko K, Shanbhag A, Killekar A, et al. Deep learning of coronary calcium scores from PET/CT attenuation maps accurately predicts adverse cardiovascular events. J Am Coll Cardiol Img. 2022;Epub ahead of print.
Disclosures
- This research was supported in part by a grant from the National Heart, Lung, and Blood Institute/National Institutes of Health.
- Pieszko was supported by a research scholarship from the Polish National Agency for Academic Exchange.
- Cedars-Sinai has a pending patent application on the use of convolutional long short-term memory for multislice medical image segmentation.
- Slomka participates in software royalties for nuclear cardiology software at Cedars-Sinai Medical Center and has received research grant support from Siemens Medical Systems.
Comments