EHR-Based CAD Risk Score Outperforms Pooled Cohort Equations at 1 Year
The findings will have implications for how EHR data can be harnessed by artificial intelligence going forward, authors say.
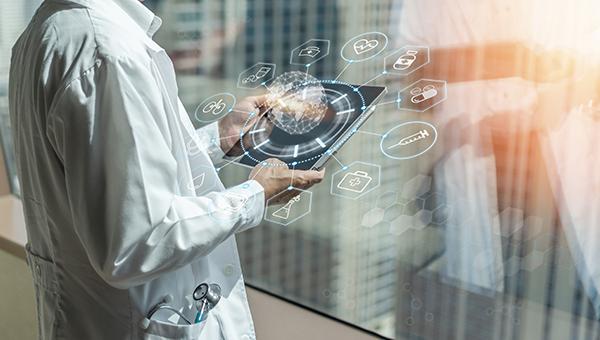
A risk-prediction score for coronary artery disease developed from electronic health record (EHR) data was as much as 12% more accurate than the American College of Cardiology/American Heart Association pooled cohort equations (PCE) at 1 year, according to new data.
Further prospective validation is needed before this score can be used clinically, but the authors say their study using machine learning to mine EHR data has implications for other areas of medicine. Until now, they note, most of this kind of research has used artificial intelligence (AI) to identify disease within images like ECG, not something as ubiquitous as medical records.
“This one of the first studies that shows that EHR has huge power to predict disease in most multi complex diseases that can be characterized as a continuous spectrum,” lead author Ben O. Petrazzini, BS (Icahn School of Medicine at Mount Sinai, New York, NY), told TCTMD. “It shows that the AI in medicine community has to take more into account medical records as a source to train models. That hasn't been really done.”
Commenting on the study for TCTMD, Rohan Khera, MBBS (Yale School of Medicine, New Haven, CT), agreed. “There has been a paucity of literature focusing on this high-dimensional data in the EHR for risk prediction,” he said. “I think this is a very important example of a study that does that in two distinct populations and then shows the benefit of that in a clear and measurable sort of way. It also shows that there is benefit in going beyond simple scores.”
Biobank Data
For the study, published in the March 29, 2022, issue of the Journal of the American College of Cardiology, Petrazzini and colleagues developed a machine-learning framework based on clinical EHR data from 555 CAD patients and 6,349 controls from the ethnically diverse BioMe Biobank and then used EHR data from 3,130 CAD cases and 378,344 controls from the community-based UK Biobank to externally validate it.
In the BioMe Biobank cohort, the EHR score in noncomorbid and random test sets improved 1-year CAD prediction by 12% and 5%, respectively, compared with the PCE. Likewise, in the UK Biobank validation population, the EHR score improved risk prediction in noncomorbid and random test cohorts by 9% and 4%, respectively. Overall, 25.8% and 15.2% individuals in each the BioMe and UK Biobank groups, respectively, had their CAD risk reclassified from what the PCE score identified. Larger improvements with the EHR score were seen in the subgroup with low CAD risk, with 20% increased discrimination and 34.4% increased reclassification in the BioMe cohort.
Also, researchers observed 14% and 23% higher positive predictive values as well as 12% and 13% improved sensitivity with the EHR score over the PCE score when CAD cases and controls from the BioMe Biobank were analyzed separately.
The polygenic risk score for CAD did not improve CAD risk prediction at all, regardless of which population or score (PCE or EHR) was used.
Different Tests, ‘Complementary’ Roles
Senior author Ron Do, PhD (Icahn School of Medicine at Mount Sinai), told TCTMD he was not sure what to expect at the outset, given that most prior work had looked at estimating long-term risk, but the “marked improvement” they observed with the EHR score in the short-term was “somewhat” surprising.
Petrazzini pointed to the EHR score’s ability to improve on the PCE score, which “tends to overestimate people that are actually not at higher risk,” he said. “We see that this model actually corrects for that overestimation.”
What he found surprising was that the EHR score did not appear to depend solely on traditional risk factors like the ones that are used to calculate the PCE score, such as blood pressure and cholesterol, but that data like hemoglobin A1c and estimated glomerular filtration rate also appeared to play a role.
Moreover, the EHR score looks at all the data as a collective and can identify risk through various interactions, not just by one risk factor alone.
“It's really about the set of features, the combination, that is important,” Do echoed. “I think it would be important for there to be further study of those set of features and how they predict cardiovascular risk. Because a lot of them aren't immediately obvious.” He added that he would also like to see this score prospectively validated before it’s used in a clinical setting.
The EHR score can say: ‘Okay, these patients may not actually be at high risk and let's focus on these other ones.’ Ben O. Petrazzini
Petrazzini highlighted that, like other risk scores, this one “is just another point of evidence for the physician to say that patients are at risk for CAD. It's not that the score is meant to actually diagnose CAD.”
But in practice, he continued, he sees the EHR score being “complementary” to the PCE score. “It could serve as a tool that automatically screens all the patients within a hospital setting,” he said. “And the fact that the EHR score is leveraging this overestimation from the PCE is important, because the PCE captures a huge amount of patients that are at risk but the EHR score can say: ‘Okay, these patients may not actually be at high risk and let's focus on these other ones.’”
Khera, on the other hand, argued that while an EHR-derived tool would be better suited to a hospital and the PCE could play a more important role in the community setting—“when you don't have a trove of data,” such as that provided by EHR—the latter doesn’t add much to the former.
Do partially agreed, noting that the EHR score “has to be confined to healthcare systems that have such data. So it could be employed as a population health screening tool in a large healthcare system where there's continuous monitoring of the score and then screening those individuals at high risk,” he said, whereas the utility of the PCE “is much broader.”
More Targeted Prevention Awaits
For the EHR-derived score to be used clinically, Khera said two things need to happen. First, the researchers need to make sure the data-derivation process is “interoperable” so that it can work with a range of different EHR types. Also, they need to be able to “account for the time varying nature of the data as it develops” by updating the data—and, hence, patient risk—as it’s captured.
Doing so could lead to better precision, Khera predicted. “Everybody should get good prevention, but the . . . usual cardiovascular prevention would be more targeted and more focused on those people,” he said.
There has been a long-standing . . . interest in pushing the frontiers of machine learning in clinical care, especially outside of the fields of image detection and computer vision. Rohan Khera
With regard to the study’s implications on AI in medicine more broadly, Khera said their methodology opens doors to using EHR data in new ways. “There has been a long-standing . . . interest in pushing the frontiers of machine learning in clinical care, especially outside of the fields of image detection and computer vision,” he said. In the past, EHR studies have been challenged by looking at individual diseases or subcomponents, but now finding “a collection of features throughout the EHR and [using] that combination in its totality” could be the way forward, according to Khera.
“The biggest advance here [is] that you don't come in with preconceived notions and downgrade the data quality by putting in clinical acumen or clinical interest on it, but actually let the data do its own teaching, of sorts, for risk prediction,” he added.
Do said he sees potential for this kind of score to be tested in other disease settings, as well, and plans to pursue that next. “I imagine . . . being able to have this continuous population health monitoring system in the EHR system that can indicate whether someone's at high risk of getting a disease,” he said.
He is also interested in being able to include specialized data like coronary artery calcium and cath lab outcomes to be able to predict “finer disease outcomes” related to CAD, like MI as opposed to general CAD.
In an accompanying editorial, Khurram Nasir, MD, MPH (Methodist DeBakey Heart and Vascular Center, Houston, TX), and Andrew DeFilippis, MD (Vanderbilt University Medical Center, Nashville, TN), write that the study gives “a peek at the possibilities of machine learning-based approaches using widely available and low-cost EHR data to ‘build a better mousetrap’ for future ASCVD risk prediction.”
Yael L. Maxwell is Senior Medical Journalist for TCTMD and Section Editor of TCTMD's Fellows Forum. She served as the inaugural…
Read Full BioSources
Petrazzini BO, Chaudhary K, Márquez-Luna C, et al. Coronary risk estimation based on clinical data in electronic health records. J Am Coll Cardiol. 2022;79:1155-1166.
Nasir K, DeFilippis A. Big data and ASCVD risk prediction: building a better mouse trap? J Am Coll Cardiol. 2022;79:1167-1169.
Disclosures
- Petrazzini reports no relevant conflicts of interest.
- Do reports being supported by the National Institute of General Medical Sciences of the NIH.
- Nasir reports receiving funding from the NIH, Esperion, Novartis, and the Jerold B. Katz Academy of Translational Research; and serving on the advisory board of Amgen, Novartis, Novo Nordisk, and Esperion.
- DeFilippis reports receiving funding by the NIH.
- Khera receives support from the National Heart, Lung, and Blood Institute of the National Institutes of Health and is a founder of Evidence2Health, a precision health and digital health analytics platform.
Comments