‘Hey, ChatGPT: Surgery or the Cath Lab?’ Study Shows AI Can Aid Decision-Making
The latest version of ChatGPT correctly made the right call 100% of the time when choosing CABG vs PCI or TAVI vs SAVR, researchers say.
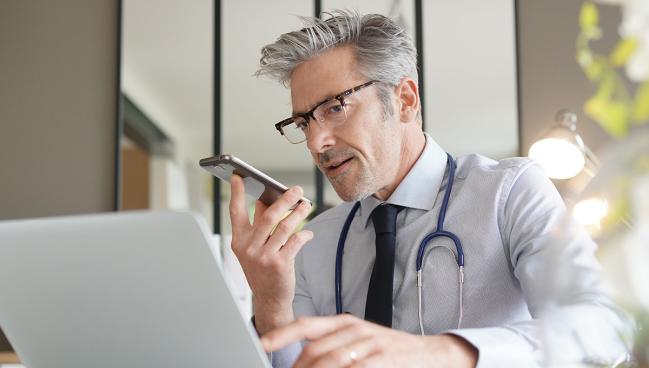
Decision-making in cardiology—specifically the choice to send a patient to either surgery or a percutaneous intervention—may one day be successfully aided by artificial intelligence (AI), according to a small, proof-of-concept study.
Large language models (LLMs) in AI platforms like ChatGPT have already been shown to be capable of generating echocardiography reports, passing clinical exams, and even authoring scientific papers. While skeptics argue against giving this technology too much power, more medical professionals now seem to accept that it will become engrained in clinical practice in the coming years, albeit with some hurdles.
“These tools will never replace the doctors,” lead author Edward Itelman, MD (Rabin Medical Center, Petah-Tikva, Israel), told TCTMD. “They will augment the role of the physician. They can help in the sphere of decision-making support systems, but it must be through [a] rigorous regulation process.”
Itelman specified that LLMs should never be making the final call, but only used as support tools. “Even the most tech-oriented patients—the most tech savvy people who trust navigation software or email clients that auto filter your email—they understand that there are mistakes,” he said. “When it comes to medicine, mistakes can have very harsh consequences.”
Many of these AI tools are publicly available, and patients are already using them to fact-check treatment plans, he said. “Patients are going to use them even more than doctors because they're very accessible, they're free, [and] they have the aura of being a little smarter than the basic Google search,” he said.
But without any regulation, as is done traditionally with drugs and devices before they become available, internet-based tools like LLMs are being used often without regard for potential repercussions, Itelman said, adding that it will be up to a collaboration between medical teams, industry, and regulators to catch up and set it on the right course.
Three LLMs
For the study, published online this week as a research letter in JACC: Cardiovascular Interventions, Itelman and colleagues asked three commercially-available LLMs in early 2024—ChatGPT v3.5 and ChatGPT v4.0 (OpenAI) and the now-discontinued Google Bard—to provide a treatment recommendation for 20 structural and 20 coronary cases according to current medical guidelines. Half of each category included characteristics making them appropriate for transcatheter intervention (TAVI or PCI) and the other half to surgery (SAVR or CABG) according to current European Society of Cardiology guidelines—all were written by interventional cardiologists with expertise in both coronary and structural interventions.
Both ChatGPT models correctly selected TAVI or SAVR in all structural cases, but Google Bard only did so 70% of the time, instead suggesting SAVR for one TAVI-leaning case and TAVI for five surgery-leaning cases.
The coronary cases proved more difficult, with both ChatGPT v3.5 and Google Bard assigning only 70% of the cases correctly to PCI or CABG. However, ChatGPT v4.0 selected the correct procedure for all cases.
When it comes to medicine, mistakes can have very harsh consequences. Edward Itelman
When the researchers asked the LLMs why they made the recommendations they did, the reasoning was often logical and mirrored current guidelines, but sometimes the answers would include outdated citations. “The use of an outdated reference is expected to be improved with newer models acquiring better citing capabilities and access to more recent knowledge,” the authors write.
“Another limitation is that, as users, we are unaware of the source of information the LLM is using,” they continue, explaining that while various societies might have different guidelines, the LLM might incorporate both without saying so. “Also, despite providing the LLM with information such as age, sex, and, in some prompts, even Syntax score and feasibility of the transfemoral approach, we know that every prompt cannot contain the complete information achievable from personally reviewing the imaging and interviewing the patient.”
Future Directions
These issues, along with others, are being addressed as LLMs evolve and are likely to be corrected with future iterations, Itelman said. Because of that, it’s only a matter of time before the technologies will become embedded within the clinical process. To move forward responsibly, he argued, “we need to establish where we stand at the moment, and how to get from this place to where we want to be.”
Importantly, Itelman said, the point of the current study is not to compare the abilities of the three different LLMs, especially since they are developing and changing so rapidly.
“Now there are newer models, and we haven't tested them and they might be better, but in the end it doesn't really matter which model is currently better or best at one specific field,” he said. “We want a large language model that is validated on most of the medical topics and the doctors and patients alike can use to glean information in a safe and secure way.”
Yael L. Maxwell is Senior Medical Journalist for TCTMD and Section Editor of TCTMD's Fellows Forum. She served as the inaugural…
Read Full BioSources
Itelman E, Vitberg G, Kornowski R. AI-assisted clinical decision making in interventional cardiology the potential of commercially available large language models. J Am Coll Cardiol Interv. 2024;17:1858-1859.
Disclosures
- Itelman reports no relevant conflicts of interest.
Comments