Novel Machine-Learning Score Can Predict FFR-Defined Ischemia Better Than Visual Stenosis
The tool predicted ischemia just as well as FFR-CT, with insights similar to those of invasive FFR and impaired blood flow on PET.
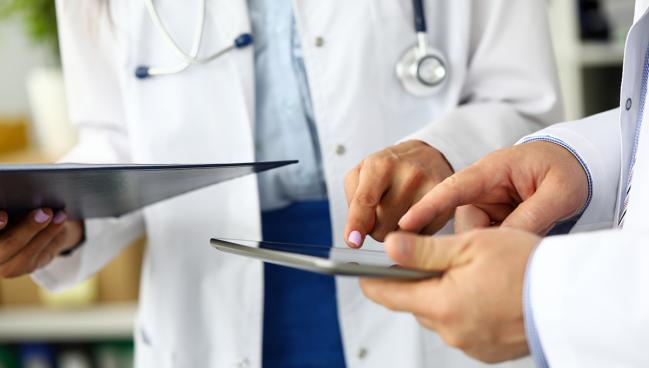
A score derived from plaque features on coronary CT angiography (CCTA) using machine learning can predict ischemia just as well as CT-derived estimates of fractional flow reserve (FFR-CT), with the advantage of being noninvasive and calculated in real time, investigators say, without the need for adenosine infusion.
According to researchers, the score outperforms standard CCTA evaluation for predicting future risk while matching the ischemia and impaired myocardial blood flow (MBF) insights provided by invasive FFR and positron emission tomography (PET), respectively. As such, they say, the score could be embedded in the clinical workflow to streamline the identification of high-risk patients before they undergo invasive coronary angiography.
“Our results are very positive,” senior author Damini Dey, PhD (Cedars-Sinai Medical Center, Los Angeles, CA), told TCTMD. “Our externally validated ML-FFR score could predict the functional significance of lesions accurately.”
The tool, as described in a paper published this month in Circulation: Cardiovascular Imaging, is currently only used for research purposes at their institution. But following regulatory approval, Dey said, “it could be applied on site immediately after plaque analysis.”
That offers advantages over currently available FFR-CT, which entails sending data offsite for analysis involving turnaround times that limit its clinical use.
She sees their score being used eventually as “a clinical decision support tool” by referring physicians before invasive coronary angiography to ascertain if the patient has a high probability of impaired FFR or a high ischemia risk. “At the same time, it can also be a deferral for downstream testing in people who have a low ischemia risk,” she said. “It would really help the efficiency and accuracy of referral to invasive coronary angiography.”
External Validation
To “train” the score, lead author Andrew Lin, MBBS, PhD (Cedars-Sinai Medical Center, Los Angeles, CA), Dey, and colleagues used data from 254 patients who, as part of the NXT trial, underwent invasive FFR in 484 vessels. The machine-learning score was then tested in 601 vessels from 208 patients with suspected CAD who’d undergone CCTA, H2O PET imaging, and invasive FFR in the PACIFIC study. Five patients and 23 vessels were excluded from the testing population due to nonevaluable CCTA scans.
In the testing cohort, 23.9% of vessels had FFR-defined ischemia and 33.6% had impaired hyperemic MBF on PET. The volumes and burdens of total plaque, as well as individual plaque components, were higher in vessels with FFR-defined ischemia compared with vessels without (all P < 0.001). The machine-learning score was better able to predict this outcome (AUC 0.92) than a visual stenosis grade (AUC 0.84; P < 0.001) and on par with FFR CT (AUC 0.93; P = 0.34). Additionally, it had an accuracy, sensitivity, and specificity of 84%, 87%, and 82%, respectively, for ischemia discrimination. Moreover, adding the score to visual stenosis grade resulted in substantial net reclassification improvement for FFR-defined ischemia (1.16; P < 0.001), driven mostly by reclassification of nonevents (82%).
The two features that were most important for predicting FFR-defined ischemia with the machine-learning score were quantitative percent diameter stenosis and low-density noncalcified plaque volume. Calcified plaque features contributed least to ischemia prediction.
In predicting impaired MBF alone, the score performed significantly better than visual stenosis grade (AUC 0.80 vs 0.74; P = 0.02) and was similar to FFR CT (AUC 0.77; P = 0.16). The accuracy, sensitivity, and specificity of the score for predicting impaired MBF were 77%, 73%, and 80%, respectively.
“Functional tools which complement CCTA without the need for additional image acquisition or drug administration have been developed to improve the detection of lesion-specific ischemia,” the authors write, citing computational fluid dynamics as one tool that be applied with currently available software. “However, this process is time-consuming and computationally expensive, requiring off-site processing by a core laboratory.” On-site FFR CT, on the other hand, is “heavily dependent on physician interaction,” they note.
“In contrast to all these techniques, our proposed machine-learning score relies only on anatomic information from CCTA without the addition of physiologic parameters, utilizes semiautomated plaque analysis, which is highly reproducible by trained clinicians or technicians, and can be computed in < 30 minutes on a standard workstation,” the authors point out.
With time, new plaque features and CCTA metrics can be added to the algorithm to improve the score and its predictive ability, Lin and colleagues add. “In this era of personalized medicine, there is a growing demand for more precise and patient-tailored risk prediction,” they conclude. A score like this “enables the interrogation of individualized predictive models by clinicians or researchers who lack familiarity with machine-learning techniques.”
Assuming the tool stands up to additional validation studies and ultimately gets the required regulatory approval, Dey said she could see the score used across the spectrum of patients who undergo CCTA. “We actually also have tested it in patients who have the first grade of coronary stenosis, so 1-25%, and there is a low ischemia risk, typically,” she said.
Dey said she feels confident in the score given the external validation they performed but acknowledged that “any additional validation in other cohorts is always welcome before it goes into clinical practice.”
Yael L. Maxwell is Senior Medical Journalist for TCTMD and Section Editor of TCTMD's Fellows Forum. She served as the inaugural…
Read Full BioSources
Lin A, van Diemen PA, Motwani M, et al. Machine learning from quantitative coronary computed tomography angiography predicts fractional flow reserve–defined ischemia and impaired myocardial blood flow. Circ Cardiovasc Imaging. 2022;15:e014369.
Disclosures
- Dey reports receiving royalties from Cedars-Sinai Medical Center and holding a patent for a method and system for plaque characterization.
Comments