Popular CV Risk Calculator Is Not Accurate Among Patients at Low Socioeconomic Standing
Living conditions and neighborhood, along with clinical factors, should be taken into account when estimating patient risk, a new study suggests.
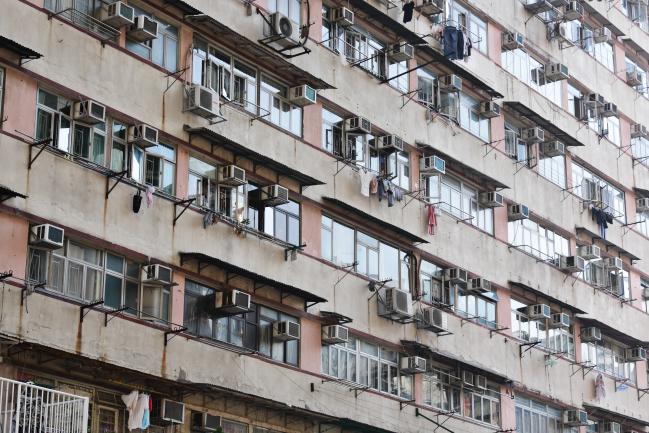
The predictive accuracy of a commonly used cardiovascular risk model is being called into question by findings of a large population-based study of patients, especially for its underestimation of risk for patients who live in disadvantaged neighborhoods.
The Pooled Cohort Equations Risk Model (PCERM) was introduced by the American College of Cardiology/American Heart Association in 2014 as a means of identifying atherosclerotic cardiovascular disease risk over 10 years. While the model has been widely incorporated into clinical practice, said lead study author Jarrod Dalton, PhD (Cleveland Clinic, OH), it lacks a key component.
“Our current assessment of cardiovascular risk relies on strictly clinical indicators,” he told TCTMD. “It might be insufficient to just look at clinical indicators in characterizing people’s risk, and we need to start to think about ways to incorporate other characteristics that aren't directly associated with the patient but might nonetheless impact risk—in particular, environmental exposures like excess stress, lack of access to healthy foods, lack of access to places to exercise, maybe some environmental exposures like air pollutants and lead contaminants, and so on.”
Published online August 29, 2017, ahead of print in the Annals of Internal Medicine, the study by Dalton and colleagues looked at 109,793 patients older than 35 years who had an outpatient lipid panel drawn at the Cleveland Clinic between 2007 and 2010. All patients were stratified by a neighborhood disadvantage index (NDI) based on several variables linked with neighborhood socioeconomic position across northeastern Ohio.
Among patients living in affluent communities, the PCERM reasonably discerned cardiovascular risk (C = 0.80; 95% CI 0.78-0.81). However, its accuracy was relatively poor for patients on the other end of the socioeconomic spectrum (C = 0.70; 95% CI 0.67-0.74). This relationship was observed systematically among several population-level analyses.
Pitfalls of Risk Mischaracterization
“If risk is being mischaracterized, then it could be possible that patients might not be being treated in an optimal manner,” Dalton said. “In fact, they might be undertreated [given] that the risk model does not capture risk in [certain] populations.”
In an accompanying editorial, Sandro Galea, MD, DrPH (Boston University School of Public Health, MA), and Katherine Keyes, PhD (Columbia University Mailman School of Health, New York, NY), write that “prediction modeling enterprise faces substantial challenges that seldom attract as much attention as they should.
“Prediction models rest on the notion that we have enough information to develop a statistical picture of a patient's future health status,” they continue. “Taken at face value, this proposition is appealing because it builds on our understanding of a broad set of risk behaviors and an emerging set of genetic and other biological markers known to be associated with disease at the population level.”
However, this study is a “sobering reminder” that there are several limitations of predictive models, Galea and Keyes argue, advising caution to clinicians who use them.
While the problem might be clear, Dalton admitted that no ready solution yet exists. “We’re still trying to grapple with optimal ways to do risk estimation in different patient populations,” but many physicians have been relying on models that they wrongly think are generalizable across all patients, he commented. “Now we have an opportunity to take advantage of increasing data sources such as the electronic health record to develop more tailored models for different patient populations.”
When estimating a patient’s risk for cardiovascular disease, Dalton advised asking questions about living conditions and neighborhood exposures that might allow a clinician to better understand how the patient might be affected outside of routine clinical factors. “Clinical risk indicators alone might be insufficient for truly modeling somebody’s risk of outcomes, and we need to go beyond the hospital walls in terms of what we can use to evaluate somebody's risk,” he said.
Yael L. Maxwell is Senior Medical Journalist for TCTMD and Section Editor of TCTMD's Fellows Forum. She served as the inaugural…
Read Full BioSources
Dalton JE, Perzynski AT, Zidar DA, et al. Accuracy of cardiovascular risk prediction varies by neighborhood socioeconomic position: a retrospective cohort study. Ann Intern Med. 2017;Epub ahead of print.
Galea S, Keyes KM. Population health science and the challenges of prediction. Ann Intern Med. 2017;Epub ahead of print.
Disclosures
- The study was funded by the Clinical and Translational Science Collaborative of Cleveland and the National Institutes of Health.
- Dalton reports receiving grants from the National Institutes of Health/National Center for Advancing Translational Sciences during the conduct of the study.
- Galea and Keyes report no relevant conflicts of interest.
Comments