Protein Risk Score Predicts Mortality in Real-world HF Patients
Though it’s still early days, there is hope that proteomics will be a new path toward individualized decision-making.
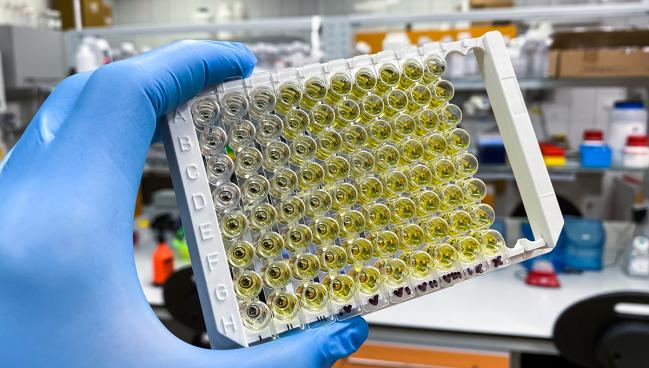
For patients with heart failure (HF), a tool that measures an array of proteins circulating in the blood can be used to predict their prognosis. The results, from a community-based study of more than 1,000 people living in the United States, are the latest to show promise for proteomics as a way to individualize HF treatment choices.
Véronique L. Roger, MD, MPH, who was senior author on the paper along with Jungnam Joo, PhD (both from the National Heart, Lung, and Blood Institute, Bethesda, MD), told TCTMD that novel platforms for proteomics, along with other “omics” tools that measure metabolites and genes, are increasingly able to handle larger and larger numbers of samples. “Here we had an assay that measured 7,000 proteins,” she said. “These are novel assays that are coming online rapidly in [recent years]. . . . These new platforms offer the advantage of scalability.”
These high-throughput tools have been used not only in heart failure but also in other forms of cardiovascular disease, cancer, Alzheimer’s disease, and other disease states, Roger noted. “It’s definitely not at all confined to heart failure.”
Yet heart failure, with its heterogeneity and complexity, and the need for timely polypharmacy, would be well suited to better risk stratification, the researchers say.
Roger said that a key contribution of the current study is that it applies the proteomics approach to a community-based cohort. Their data source, a three-county registry conducted in southeast Minnesota, “was really designed to represent a community practice in a very specific geographical area in the United States,” she explained, adding that this design bolsters confidence that their results would apply in the real world, or at least in that particular setting.
A 38-Protein Score
Led by Kayode O. Kuku, MD, and Joseph J. Shearer, PhD, MPH (both from the National Heart, Lung, and Blood Institute), the researchers studied a total of 1,351 patients with heart failure who were enrolled in the Rochester Epidemiology Project between 2003 and 2012.
The median patient age was 78 years, 48% were women, 29% had an ejection fraction < 40%, the median MAGGIC score (which uses 13 clinical variables to estimate mortality in HF patients) was 25, and the median NT-proBNP level was 8,903 ng/L. Follow-up lasted through 2021. During this time, 1,013 patients died. The 5-year mortality rate was 52.1%.
The team used the SomaScan Assay (SomaLogic) to analyze 7,289 plasma proteins, ultimately creating a risk score based on 38 unique proteins. These included renin, epidermal growth factor receptor, growth/differentiation factor-15, collagen alpha-2[XI] chain, renin, NT-proBNP, cartilage intermediate layer protein, and interleukin-1 receptor-like 1, among others.
In the study’s development cohort (n = 855), after adjustment for MAGGIC score, high risk scores were linked to higher mortality (HR 2.62 per 1-SD increase; 95% CI 2.34-2.93). Risk stratification improved with further adjustment for NT-proBNP. Results were consistent in the validation cohort (n = 496).
Moreover, the estimated to observed mortality ratio using the protein risk score was 1.01 (95% CI 0.92-1.10), superior to the ratio obtained with clinical models alone (1.01; 95% CI 0.92-1.10). Calibration also worked well at gauging risk at both the lower and higher extremes, and for both reduced and preserved ejection fraction.
Adding the protein risk score to the clinical model reclassified patients such that more were in the lowest-risk group (5-year mortality risk ≤ 25%) and more were in the highest-risk group (5-year mortality risk >75%) than when using the clinical model alone.
Incremental Gains
Joseph G. Rogers, MD (The Texas Heart Institute, Houston), commenting on the findings for TCTMD, agreed they hold promise. “This is an interesting paper that adds to our ability to risk-stratify heart failure patients,” he said in an email, pointing out that the population has a good representation of both sexes and includes a majority with preserved ejection fraction.
“I always worry about whether new risk prediction models will incrementally improve a clinician’s ability to stratify risk for an individual patient or a population,” said Rogers. Here, the researchers used several techniques to try to overcome that concern, looking at how the model performed alongside the commonly used MAGGIC clinical model and NT-proBNP biomarker as well as doing a net reclassification of risk. “All appear to incrementally improve our ability to stratify risk in this population,” he agreed.
As to the specific proteins of interest, “we are continuing to refine our understanding of heart failure biology,” Rogers explained. “Some of the dysregulated proteins are not new in risk prediction models whereas others are less obviously linked to the condition. This type of analysis may ultimately provide new therapeutic targets.”
Kuku et al say a score that’s able to accurately predict mortality risk could be used in clinical decision-making to pinpoint who might benefit from referral to an advanced HF specialist, rapid drug titration, mechanical circulatory support, and/or transplantation.
“Our results provide the proof of concept that proteomics can address this need with greater precision than current clinical methods. By illustrating the potential of high-throughput omics to improve the clinical management of the HF syndrome, our findings are directly relevant to clinical practice and strongly support the pursuit of the evaluation of proteomics for this purpose,” they conclude, adding that a potential limitation of their study is that its participants were mainly of European descent.
To TCTMD, Roger said much research lies ahead. For example, it would be interesting to look at a control group without heart failure, or differences among individuals with various types of heart failure. “What makes research so exciting is that it provides some answers but also raises a lot of other questions,” she commented.
With genomics, thanks in large part to insights from the Human Genome Project, genes are now being used to guide breast cancer therapy, but it took years for that technology to evolve before it could be used in clinical practice. “One can think about a similar paradigm for heart failure. As we become more used to these platforms, as we understand better their capabilities, and as [the tests] themselves become cheaper, . . . one can assume reasonably it will happen,” she said, that they will inform heart failure care. How long that will take isn’t known, she cautioned.
Even so, it’s not too early to think about how having the ability to better predict prognosis in HF patients would impact care, observed Roger. “Regardless of the tool that is used to [do that], a better calibration of the prognosis is critical to make clinical decisions,” so it would be welcome.
Caitlin E. Cox is News Editor of TCTMD and Associate Director, Editorial Content at the Cardiovascular Research Foundation. She produces the…
Read Full BioSources
Kuku KO, Shearer JJ, Hashemian M, et al. Development and validation of a protein risk score for mortality in heart failure: a community cohort study. Ann Intern Med. 2024;Epub ahead of print.
Disclosures
- The study was supported by the Division of Intramural Research at the National Heart, Lung, and Blood Institute of the National Institutes of Health. It also used in part the resources from the Rochester Epidemiology Project medical records linkage system, which is supported by the National Institute on Aging, the Mayo Clinic Research Committee, and fees paid annually by Rochester Epidemiology Project users.
- Kuku, Shearer, and Roger report no relevant conflicts of interest.
Comments