SWIFT TAVI Algorithm Lowers Wait Times for Highest-Risk Patients
The simple tool includes just three factors, making it easy to use by the nonclinical staff most likely to use it.
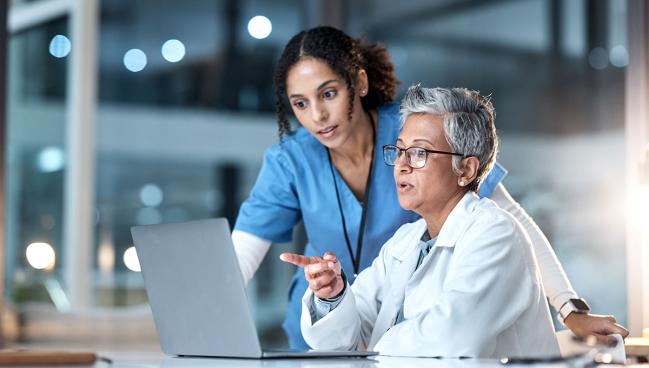
PARIS, France—Using a simple, automated algorithm can reduce wait times for TAVI without increasing rates of morbidity or mortality, according to a new analysis.
“For us, this was drawn out of clinical concern and need, because our TAVI waitlist times were burgeoning,” said Sarosh Khan, MBBS (Essex Cardiothoracic Centre, Basildon, England), who presented the findings here today at EuroPCR 2024. “We said: we need to come up with a pragmatic solution that is easy and simple to apply to our patient population and our waitlist, so that we can get an estimated clinical risk and prioritize accordingly.”
In the United Kingdom, Khan explained, National Health Service (NHS) policy had dictated that while patients referred to TAVI were usually seen in clinic within 2 weeks, their TAVIs were scheduled solely by the order of their clinic dates without regard for any other risk factors or urgency.
They developed the SWIFT TAVI algorithm, which includes just three scored variables—LVEF (0-3 points), peak AV gradient (0-5), and presence or absence of syncope (0 or 10 points)—and grades patients according to their final clinical risk profile:
- Lowest: 0-1 point
- Low: 2-3 points
- Intermediate: 4-8 points
- High: 9-10 points
For the study, Khan and colleagues included 228 patients (mean age 82 years; 60% male) who underwent TAVI at their center, including 111 controls treated per usual in 2021 and 117 who were scheduled with prioritization based on SWIFT algorithm in 2022. All patients were classified by the SWIFT for the purposes of the study, with about 10% considered lowest risk, 15% in each of the low and intermediate risk categories, and the remaining 60% high risk.
Compared with standard allocation, using the SWIFT tool to prioritize TAVI scheduling significantly reduced wait times for intermediate-risk patients from about 20 to 10 weeks (P = 0.014) and for high-risk patients from about 32 to 12 weeks (P < 0.001).
Moreover, the rate of MACE for patients waiting for TAVI remained similar before and after the researchers began prioritizing TAVI based on the algorithm (9% vs 10%).
“We never set out to try and reduce mortality rates because we knew we wouldn't have the numbers to achieve that or the power,” Khan told TCTMD. “But what we did want to make sure was [that] our tools shouldn't increase it.”
Further, patients in the high- and intermediate-risk categories were the ones for whom they were most interested in reducing wait times, he explained. “But the intermediate- and low-risk are the vast majority, [and] we don't want them to pay a penalty at the expense of the highest risk. And that we didn't see.”
Which Factors to Include?
Commenting during the session, Mohamed Abdel-Wahab, MD (Heart Center Leipzig, Germany), said that the TAVI waitlist at his institution is “not that long, around 3-4 weeks.” Even so, he noted, “every now and then we struggle, of course, with how to identify patients that still cannot wait for these short periods of time.”
The selection of the right parameters to pick for prioritization is tricky, and Khan noted that the ones they choose were included purely for pragmatic purposes. Some on the discussion panel suggested also including NYHA class and other biomarkers, but, he said, “the more variables you add, the more resistance you get to the applicability and ease of use, and therefore the pragmatism will be lost. So it's finding that fine balance of the correct variables and getting the best outcome.”
Khan stressed that in their experience, nonclinical staff members have been the one using this algorithm and scheduling patients. “They need variables that don't need interpretation, are easier to obtain and then to apply,” he said.
Further Refinement
Commenting during the session, co-author Christopher Cook, MBBS, PhD (Essex Cardiothoracic Centre), said they faced some initial resistance with using the algorithm, but now their team relies upon it. “From an IT point of view, our nurses didn't feel confident doing it,” he noted. “But it's now fully automated and they cannot live without it, because they're no longer having to remember one particular patient was very unwell or the ejection fraction of this patient wasn't good.”
That said, he acknowledged that the team also doesn’t want lowest-risk patients “always being pushed to the end of the queue.” Their system has a “hard endpoint” of 16 weeks, after which low-risk patients are prioritized for their procedures, Cook explained.
Khan said they are currently starting to use a second iteration of the SWIFT tool that weights different factors as well as wait time. He hopes to be able to present more data from this version next year. Additionally, he’d like to see future versions of the algorithm incorporate frailty and look to see whether using this kind of prioritization shortens length of stay.
For now, he said the SWIFT TAVI algorithm is ready for use, even outside of the UK, according to Khan. Rural regions in the US or other areas in Europe with longer waitlists, he suggested, could stand to benefit by prioritizing patients with this algorithm. “It's very easy, it's platform agnostic, [and] it's a Microsoft Excel program. You input your values and you get an automated outcome, so your waitlist gets dynamically adjusted as you go through,” he said.
And this is important because waitlists will only continue to grow as TAVI becomes indicated for more and more patients as well as with the growing number of TAV-in-TAV procedures. “The demand is going to exponentially increase,” Khan said. “You can increase the number of operators, but then if your facility cannot handle downstream—overnight stays, bed stays—that is a significant burden as well.”
Yael L. Maxwell is Senior Medical Journalist for TCTMD and Section Editor of TCTMD's Fellows Forum. She served as the inaugural…
Read Full BioSources
Khan S. Reducing TAVI waitlist times using an automated algorithm-driven prioritization score: SWIFT TAVI algorithm. Presented at: EuroPCR 2024. May 16, 2024. Paris, France.
Disclosures
- Khan reports no relevant conflicts of interest.
Comments