‘Digital Biomarker,’ Derived From EHR, Can Diagnose and Describe CAD
Using AI to describe the spectrum of disease could have implications for delivery of precision medicine, researchers say.
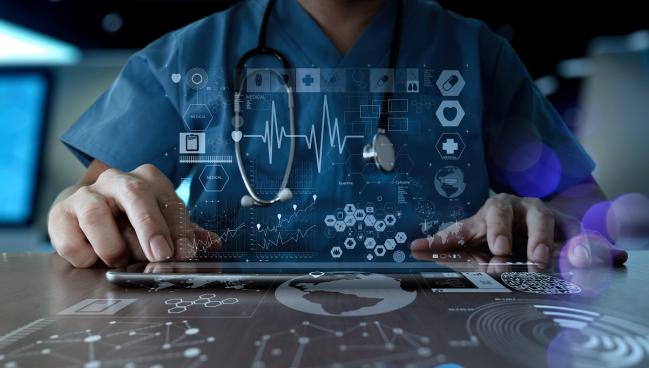
A new machine learning-derived “digital biomarker” has the potential to not only diagnose CAD from electronic health record (EHR) data but also characterize its features and the risks those entail.
“Prior to this work, machine-learning studies have been used to predict CAD on a case-control fashion as a binary disease,” meaning the disease is either present or absent, senior study author Ron Do, PhD (Icahn School of Medicine at Mount Sinai, New York, NY), told TCTMD. “None of these studies have looked at using CAD on a spectrum of disease, despite prior studies showing that the disease exists on a spectrum.”
For example, even a study published by the same group earlier this year showing that a machine learning-based score outperforms the pooled cohort equations for CAD risk assessment at 1 year was limited in its ability to quantitatively describe the characteristics of disease, Do explained.
“Diseases are not just neat boxes of ‘you have disease’ or ‘you don't have a disease,’” lead author Iain Forrest, PhD (Icahn School of Medicine at Mount Sinai), said. “It's messy and we wanted to better capture that.” Also, he noted to TCTMD, the plethora of “passively collected” EHR data available in most health systems is still an underused resource.
“We lose information when we characterize individuals into discreet groups of CAD cases or controls,” Do further explained. “By building or constructing a quantitative marker of CAD, we show that this quantitative marker is associated with different gradations of risk for various clinical outcomes.”
Broadly, this kind of tool has the potential to be applied to other types of cardiovascular disease, as well. “You could target all sorts of diseases, metabolic diseases, and different physiological diseases,” Forrest explained. “Our aim is to apply this method and this way of reconceiving diseases and researching in the clinical space as a spectrum for other diseases as well.”
ISCAD Results
For the study, published online recently in the Lancet, the researchers included EHR data from 35,749 and 60,186 participants in the BioMe Biobank (median age 61 years; 41% male; 14% with CAD) and UK Biobank (median age 62 years; 42% male; 14% with CAD), respectively. They trained their 0-to-1 ranging model, which they called in-silico scores for coronary artery disease (ISCAD), on a segment of patients from the BioMe cohort, tested it on the rest, and then validated it using the UK Biobank data.
Using known risk factors, pooled cohort equations, and polygenic risk scores, the ISCAD model was able to predict CAD well, with high sensitivity and specificity in all three test sets.
ISCAD Prediction Among Test Sets
|
AUC |
Sensitivity |
Specificity |
BioMe Training |
0.95 |
0.94 |
0.82 |
BioMe Holdout |
0.93 |
0.90 |
0.88 |
UK Biobank Validation |
0.91 |
0.84 |
0.83 |
Additionally, as ISCAD quartiles increase per 12 percentage points, so did the risk of quantitative disease characteristics—like obstructive CAD, multivessel CAD, and stenosis of the major coronary arteries—as well as outcomes like all-cause death and MI.
Interestingly, the researchers identified 12 individuals (46%) with high ISCAD scores (≥ 0.9) and clinical evidence of CAD who were undiagnosed.
While the population used to train ISCAD is fairly diverse, both Forrest and Do say they’d like to see further validation using equally, if not more, diverse test sets. This could potentially lead to building ethnicity-specific ISCAD scores, they say. Also, prospective studies to test whether making clinical decisions based on ISCAD has an effect on outcomes are warranted.
From there, Do said their team is also working on developing “a more portable version of the score” using a more limited set of clinical data points that would be available in all health systems, hence broadening its applicability.
All of this is leading toward achieving precision medicine, Forrest said. “It really is like kind of a holy grail to be able to obtain some sort of measurement that can help distinguish these nuances and different complexities between patients that have a certain disease,” he said. “That's why I think we were very surprised at not just how well this digital marker was able to stratify the amount of plaque inside a patient's arteries of their heart, but also all these other very important facets of the disease.”
Another application of this kind of tool would be in helping recruit specific patients for clinical trials, Forrest continued.
Clinical Applications, Future Directions
In an accompanying editorial, Puneet Batra, PhD, and Amit V. Khera, MD (both Broad Institute of MIT and Harvard, Cambridge, MA), write that identifying patients on a spectrum of CAD, as opposed to how today’s available risk scores are based simply on coronary anatomy, “could enable tailored interventions that would be better aligned with coronary artery disease risk.”
It's messy and we wanted to better capture that. Iain Forrest
To TCTMD, Batra said he foresees two optimal uses for ISCAD in clinical practice. “First is identifying preclinical cases in the healthcare setting where you can start applying preventive therapies. Maybe there is really subtle evidence of coronary artery disease that a physician would miss, and a model like this could help a physician realize that,” he said. However, because EHR data are “messy,” with information known to the physician that is not always collected, Batra said there could be “ascertainment bias.”
“I worry a little bit that models trained on . . . this kind of physician-reported data collected in a hospital setting [are actually] measuring what the physician already suspects, as opposed to learning something that nobody knows,” he explained. “It is just something to be cognizant of.”
The second potential use for ISCAD could be that “it opens the door to precision care,” according to Batra. Using the information from it to guide prescription or screening decisions in patients with known disease “can help guide treatments more accurately,” he noted.
Batra said he would also like to see this model externally validated in other populations and cautioned against “overhyping” it before prospective data becomes available. “This is such an important contribution if it turns out to be true, so we should follow it,” he said. “There are a lot of lives that could be affected by this.”
Stressing the latter, Batra said: “There are a lot of minority groups and diverse groups that do not get a lot of attention. These models can actually work better for everyone if you train them on those groups.”
Yael L. Maxwell is Senior Medical Journalist for TCTMD and Section Editor of TCTMD's Fellows Forum. She served as the inaugural…
Read Full BioSources
Forrest IS, Petrazzini BO, Duffy Á, et al. Machine learning-based marker for coronary artery disease: derivation and validation in two longitudinal cohorts. Lancet. 2022;Epub ahead of print.
Batra P, Khera AV. Machine learning to assess coronary artery disease status—is it helpful? Lancet. 2022;Epub ahead of print.
Disclosures
- Do reports receiving grants from AstraZeneca; grants and non-financial support from Goldfinch Bio; being a scientific co-founder, consultant, and equity holder for Pensieve Health; and being a consultant for Variant Bio, outside of the submitted work.
- Forrest reports no relevant conflicts of interest.
- Khera reports receiving grants from IBM Research during the conduct of the submitted work to research the topic of machine learning in cardiovascular disease; as well as receiving personal fees from, employment by, and equity in Verve Therapeutics, and personal fees from Amgen, Novartis, Silence Therapeutics, Korro Bio, Foresite Labs, Third Rock Ventures, Color Health, Veritas International, Sarepta Therapeutics, and Ambry, outside of the submitted work.
- Batra reports receiving grants from IBM Research, grants from Bayer, personal fees from Prometheus Bio, Flagship Pioneering, Recursis, and Novartis, outside of the submitted work.
Comments